
Self-Service Data: 5 Ways to Scale to Real-World Conditions
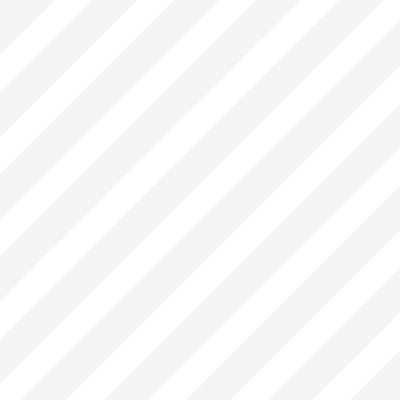
Self-service analytics is a necessity for many organizations, for the simple reason that there is no central data analytics group large enough to scale to the needs of the business. My last two companies, which have employed 7,000 and 150,000 people, respectively, are two cases in point.
But good self-service data strategy happens in phases, with these phases corresponding to the maturity of the organization’s analytics capabilities, as well as the data literacy maturity of the employee base.
Symptoms of self-service immaturity
Common challenges you may experience with self-service analytics include consistency of the analysis, as well as the relevance and accessibility of the data. Imagine a mid-level manager who has a disparate, possibly outdated data set on their own personal computer. They do some analysis and send out the results to their team. This presentation ends up on the desk of a senior leader, and before you know it you’re spending a bunch of work cycles on findings that are outdated in the best case scenario, and more likely wrong or misleading.
Here are five principles I’ve learned to follow to enable self-service analytics in a way that benefits the whole organization:
- Build easy-to-use analytics tools. I think of my data team as a product management team. In other words, we create products with a measurable life cycle relevance that help to address the most commonly asked questions of the business. Creating our own tools means we can control the environment, but that people can still interpret data using different filters relevant to them. Most important, with our tools they’re using relevant and near-realtime data or realtime data to do their analysis.
- Allow for some user flexibility. Until recently I was hesitant to authorize exporting data outside of our self-service tools, but once I realized we had a controlled environment, we could answer 95 percent of the questions being asked. Users needed to be able to export the data so they could drill down into it. Accepting that was a good decision, because we removed another barrier for users. They weren't getting a large global dataset, because access was role-based, but they could explore and drive more relevance.
- Create an accessible path for help. Self-service analytics shouldn’t mean you’re on your own. Data leaders can consider building an avenue or path back to their Center of Excellence team when users need something beyond the basic tools that they couldn't develop on their own. Offering this assist also allows you to prioritize and track requests.
- Train everyone in data storytelling. I strongly believe that every user needs to be able to tell stories with data, whether that’s to an internal customer or an external client. It’s never about the spreadsheet or the dashboard; it’s about what that’s telling you, why you should care, and what you should do about it. That typically requires some training.
- Measure tangible and intangible impact. As the saying goes, Measure what you can manage, and manage what you can measure. Tangible measures of success tend to track to the product management life cycle. For example, what level of engagement are our tools generating? How often are users going to them? What's the churn rate? Do I have people who show up one week or month, but don't ever come back? Is use rising over time, or has it stalled? On the intangible side, engage with managers and ask, Is the way your employees present business cases more supported with data? Are people using data more effectively to tell a story? Is better data showing up in Quarterly Business Reviews with customers?
Why are these steps so important for self-service analytics? I think my teams have tired of me repeating this sentiment – “This is not Field of Dreams. If you build it, they may not come.” – but I keep saying it because I want it to be a mantra in their work. Success is not only about having great tools to answer questions. It’s about teaching users why they should use these tools, and why the decisions they make will be stronger as a result.
When it all comes together, you may have fewer conversations like the one with the manager who brings their own data (and misleading findings), and more like this one I recently had:
“I've got the access to the data. I now know how to tell the story. Here's my
before and after. Here's what I would've shown last month, and here's
what I'm showing now.”
There's nothing more gratifying for me than providing people with data and the tools to use if effectively. I can't precisely measure outcomes like the enthusiastic response from the employee above, but I realize as a clear sign that our self-service analytics are heading in the right direction.
I think of my data team as a product management team. In other words, we create products with a measurable life cycle relevance that help to address the most commonly asked questions of the business.