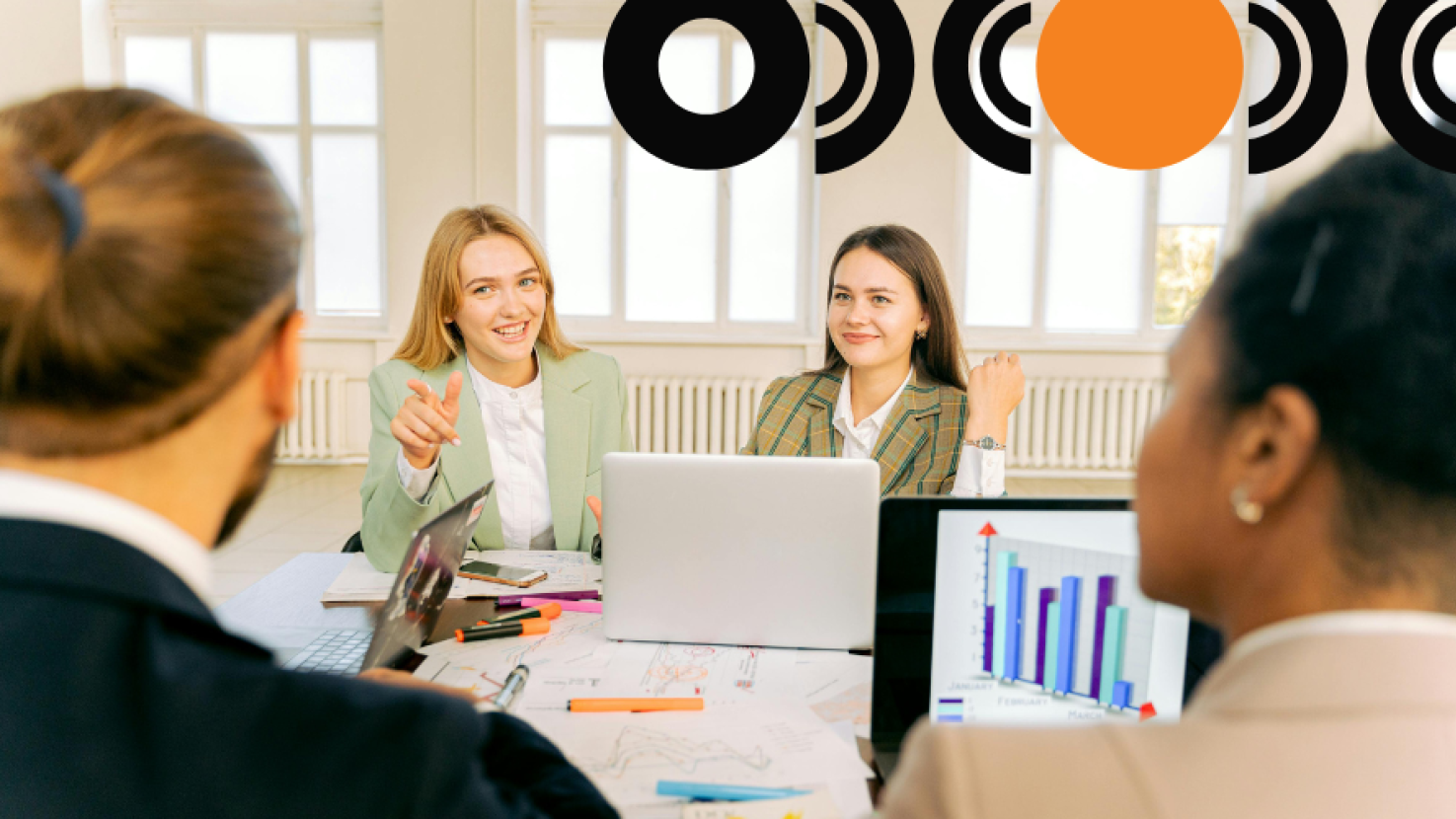

5 Tips for Data-Driven Innovation in Business
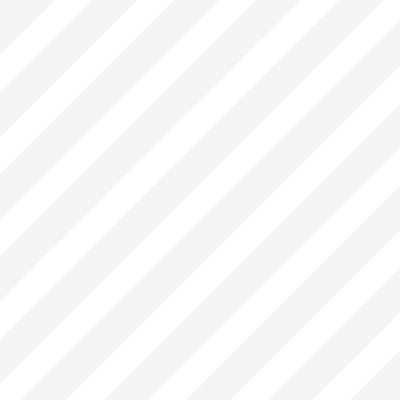
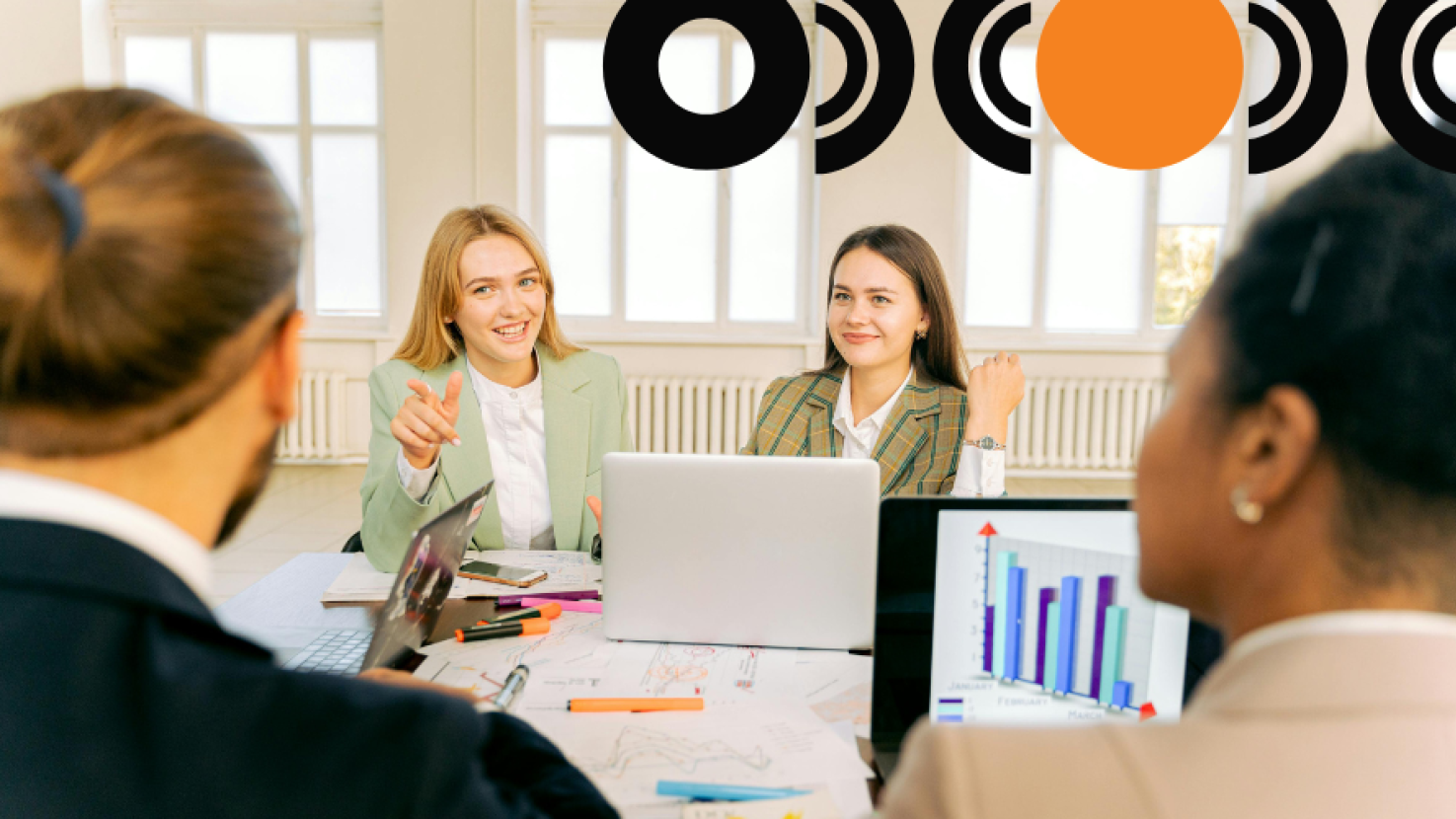
A recent statistic from Gartner that has stuck with me claims that 65% of organizations use data and analytics primarily to validate decisions they’ve already made. As someone who’s always striving to use data more proactively, this raises a pressing question: How should organizations be using data to drive innovation rather than confirming what they already know?
To me this is a classic ‘Ready, Fire, Aim’ problem. It typically begins when someone has a pre-cast data analytics solution and needs to find a problem or fix, almost as a proof of concept for the solution. It’s a natural bias, of course, and more common than you might think.
Yet rather than trying to combat this bias, I have been exploring more controlled experiments. This involves leaning into leadership engagement and helping channel it into trials powered by data that we can validate differently.
5 innovation use cases for data
Here are some powerful use cases I’ve seen over the past year for how data-driven innovation can be utilized as a competitive advantage rather than having data be deployed to reverse-engineer a decision that’s already been made.
- Cross-Solving. In my business group we are working hard on transitioning from a traditional, reactive break-fix approach to customer support to one that is more proactive and consultative. Many SaaS companies today have great insight into their customer base that they can use to “cross-solve” unreported technical issues. It’s a bit like a bank cross-selling you new products while they have you on a call for something else. Leveraging data proactively can allow you to say, “While I have you on the phone, I see that you may have another issue you haven’t asked me about – would you like to resolve that now, so you don’t experience a potential issue in the future?” Who wouldn’t want to do business with that organization?
- Personalization. We have several customer experience principles that we live by every day. One of them is to treat our customers like a person, and not just another number, service request, or sales opportunity. In order to do this effectively, we need to make sure we understand who they are and what their situation is. Data can help fill in those contextual gaps and make their experience one that feels (and is) real.
- Self-Service. A great example of a Generative AI use case is to deliver more self-service capabilities to a customer. Like a lot of people, if I have a problem with my coffee machine or phone I start with an internet search to see if I can fix it myself without the hassle of speaking to someone on the phone or through an online support portal.
The enterprise customer experience should be no different. By using data, we can create more self-service help or even GenAI chat capabilities to help customers validate their problem and identify known solutions they can implement – without ever having to reach out to a support agent.
- Process Optimization. How many times have you been on the phone when someone transfers you to another person, and you have to state your problem over again, answering the same questions? It shouldn’t have to be like this. GenAI can review all of the internal notes or customer-provided information and create a summary of events. This makes it easy to transition a case or opportunity to another team member without losing important customer context, and without losing speed to resolution.
- Conversational analytics. I recently heard a CIO say, “Dashboards are old technology.” And I think he’s right: getting too focused on dashboards as the ultimate output mistakes the forest for the trees. With new GenAI capabilities, we can evolve to more conversational analytics that answer specific questions we have versus outputting a dashboard, presentation, or chart that puts the onus on users to find the answers
Fighting process complexity with data innovation
Recent studies among engineers have shown that products continue to become more complex, and that managing this complexity requires new tools. What role can data play to help simplify this environment while enabling the creation of more innovative products?
My observation is that yes, today’s products are adding more features and functionality all the time. But the real complexity often exists within different integrations or configurations and customizations. Data and analytics tools can help identify potential performance issues when it comes to these interconnections and identify where bottlenecks may exist that are limiting the solution effectiveness. If your applications are so customized that you can’t absorb quarterly or semi-annual functionality updates from your SaaS vendor, you’re falling behind. That’s why a customer recently told me that companies should “learn to love vanilla.”
Using AI and ML can improve business process management and optimize workflows to ensure that a technology needs fewer customizations. With AI in the picture, organizations don’t need to ‘lift and shift’ technology from one platform to another while keeping the same workflows. Why drop a high-performance engine into your car but not update your balding tires? Using AI tools you can get the full benefit.
A new era in data-driven innovation
Overall, I think we’re beginning to bend the curve of using data to justify decisions rather than confirm our observations. I expect that use cases will begin to build and manifest themselves at a faster rate during the next 18 to 24 months.
Of course, all of this innovation-centric activity must be governed by security, privacy, and ethical behavior. Customer data is the most privileged of all data, and should be highly secured, either restricted completely or only granted access on specific and agreed-to use cases. Once you’re done with it in a specific use case, it should be purged.
As data leaders we also should be using data to track adoption and do benchmarking. Is this team ahead of the curve or behind it? What should this other team consider next on their innovation journey?
Finally, we should be going all in on prompt engineering. How can we engineer questions with generative AI to make sure that we get repeatable, relevant, and accurate results every time? I think this combination of good use cases and better prompt engineering will dramatically expand the applicability of AI for you and me in everyday activities in our business.
Now that we’re beginning to become comfortable with the requirements for successfully applying GenAI, I think a whole new world of innovative possibilities is within our reach. We just need to orient ourselves to the future rather than the past.