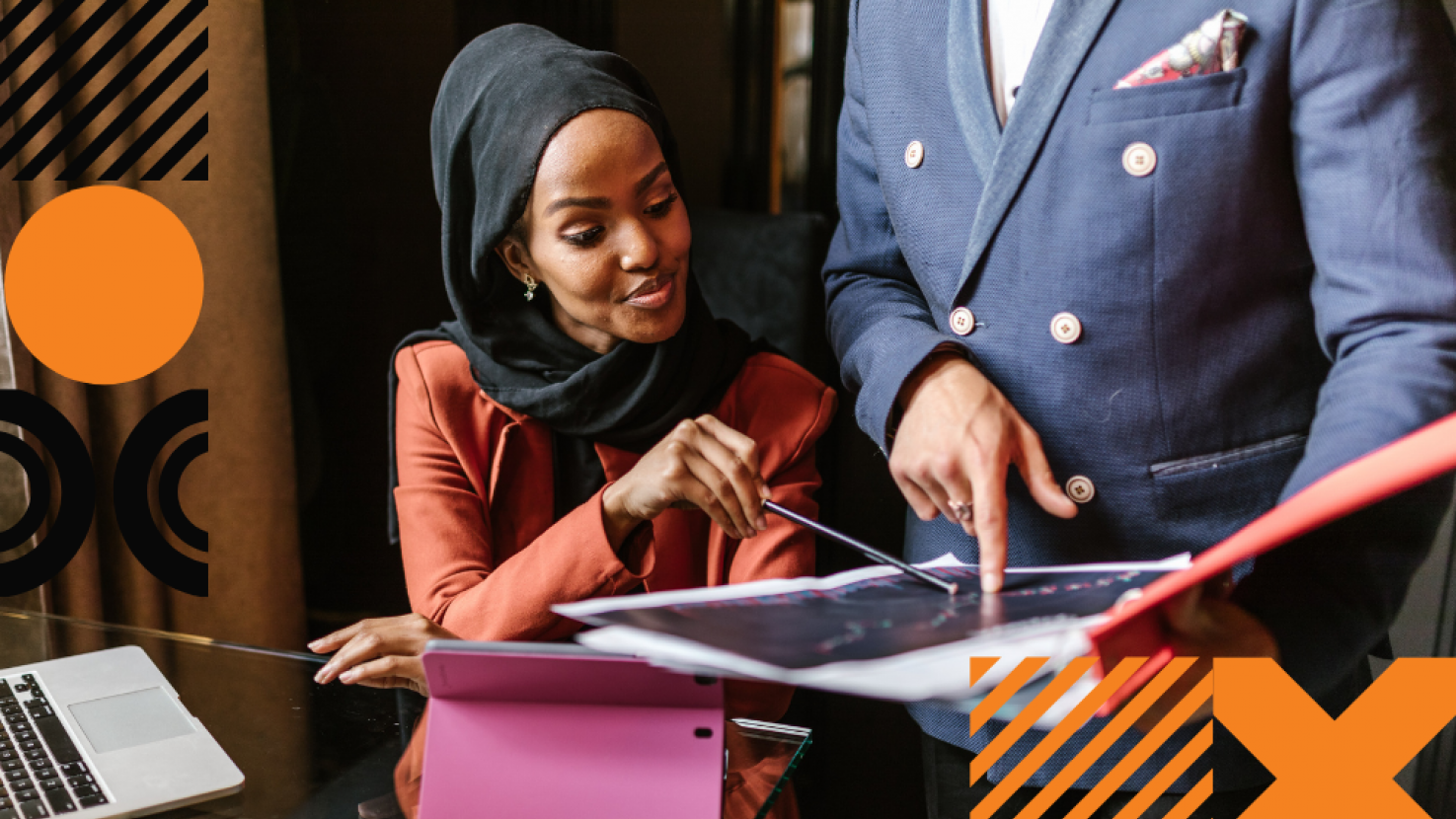

AI Ethics and Regulation: A Guide for CDOs
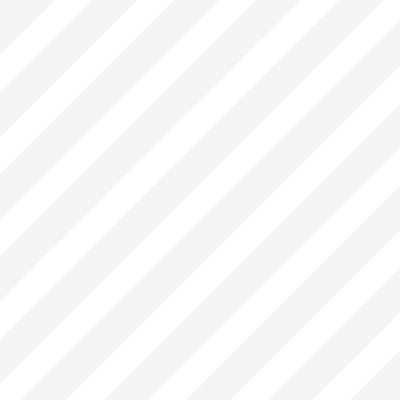
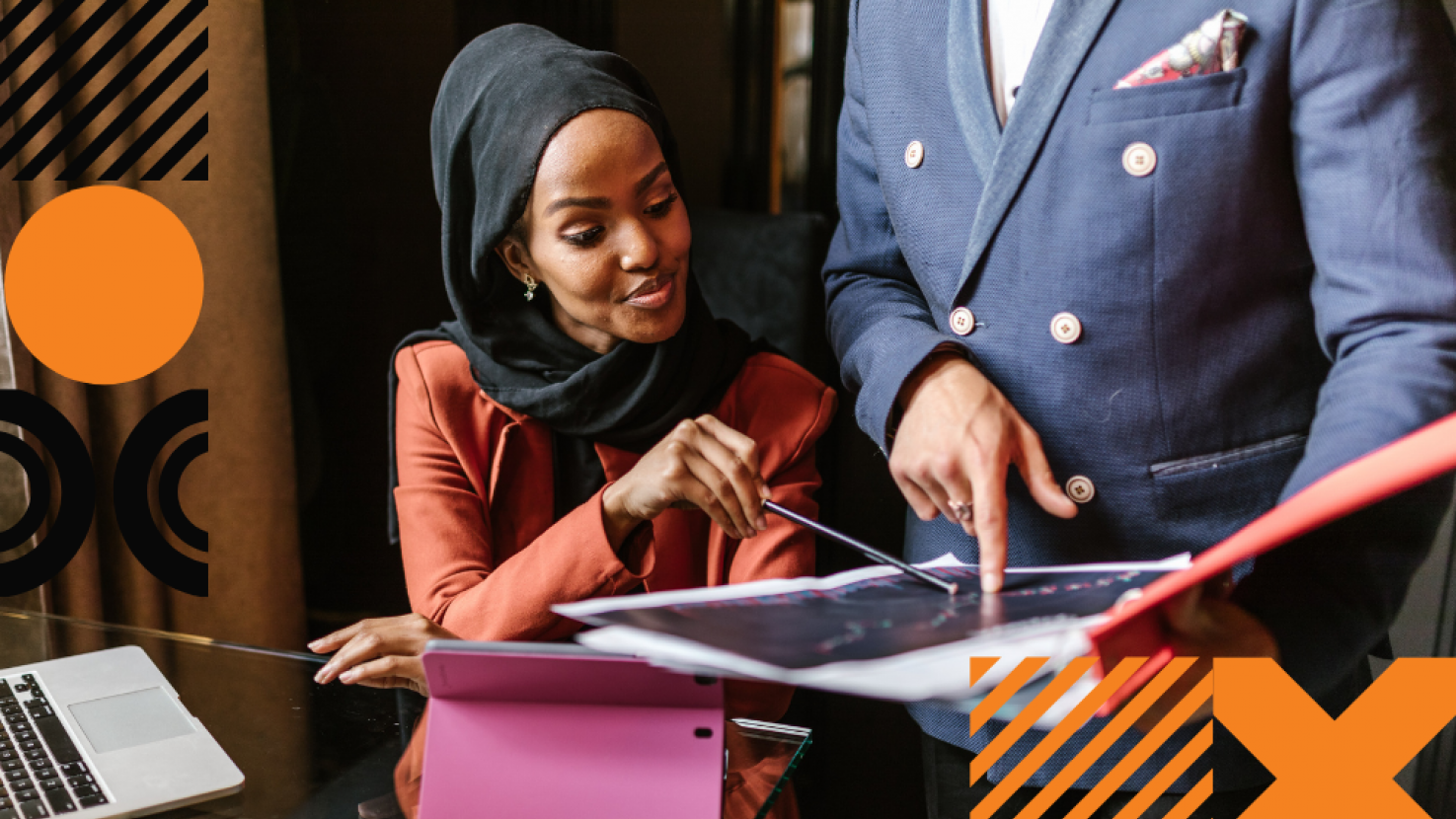
How can a data leader respond to the ethical implications of artificial intelligence (AI) if they can’t see those implications? Most data leaders are flying blind with respect to the ethics of AI in two ways, both of which can be solved with a shift in thinking.
Let’s start with the challenge: a 2021 NewVantage Partners study on data and analytics reveals that less than half of enterprise leaders feel they have strong policies to govern data and AI ethics, and barely a fifth believe the industry has done enough to address these issues.
In the same study, 91% reported they’re investing more in AI.
So, firms are investing more in AI, yet few have a handle on managing it. We’ve got work to do!
The AI wild west
It’s not all hype. AI is spreading like wildfire because there’s so much to gain. From generative AI programs like Midjourney to real-time AI-driven healthcare in Singapore that predicts disease a year early, the benefits are proving to be profound.
As with so many novel technologies, AI presents much to lose as well. The dark side of AI lies in its potential for harm, bias, and risk. These are questions of ethics, and two issues that lie at the root of the AI challenge. First, behavioral economics research has revealed that humans can’t detect their own biases. Since humans create AI, algorithms inherit the bias of their creator. AI ethics is not only complicated, then it’s hard to even see. Second, most organizations haven’t begun to govern AI yet, and some don’t even know where to look.
As a result of all this promise (and potential peril), we’re living in a bit of an AI Wild West right now as it pertains to the ethics of AI. That’s why regulators are moving to put policies into place.
The new data elephant in the room
AI brings a new player to the data game: data scientists. And unless you really loved statistics in college, it’s tough to decipher what some data scientists are talking about. After all, who dares question someone who is artificially intelligent?
Data leaders must reach out and embrace data scientists, because the algorithms they create must be first trained on data, then deployed into production with the right operational data so that algorithms may be used to make business decisions. Embracing data science teams adds another group and set of requirements to an already full CDO (or similar data leader) plate.
Behavioral economics shines a light on bias blind spots
Uncovering bias is one of the most interesting questions facing the use of AI because it takes social science and computer science to solve it. As Pulitzer Prize-winning behavioral economist Daniel Kahneman suggests in Noise: A Flaw in Human Judgment, reducing bias is hard, because humans can’t spot their own biases.
Compounding the human blind spot problem is a technology problem. Data science teams often pass algorithms to IT who enshrine them in code. Once embedded in this way, it’s easy to lose sight of intent. Furthermore, the efficacy of algorithms tends to “drift” as conditions change, making it essential to re-train and re-calibrate algorithms.
Tools can’t mitigate bias by themselves. Bias is in the eye of the beholder. So the key to shining a light on AI bias are what Kahneman calls Decision Observers, or team members armed with checklists that screen algorithms for unintended bias and risk.
By properly governing the technical artifacts of AI, decision observers can see, analyze, and adjust their assumptions. And by employing Decision Observers, properly managing algorithms, and encouraging collaboration, we form a platform for more effective AI with fewer ethical issues.
Of course, we can’t forget the data, either. I recently met with the CDO of a top-tier bank who had no idea how their data science teams were using their data. All this person knew is that they wanted it. That’s a big red flag. Data and algorithms are teammates, and must be considered as one.
Enter the EU AI Act
The EU Artificial Intelligence Act is a European proposal that defines an ethical framework for AI applications. Although it’s not yet law, its framework and principles are in many ways what organizations have been asking for when it comes to guidance about the ethical questions surrounding AI.
What I love about the AI Act is its balance between humanistic, common-sense principles and technology. It is not about fines and bright lines, which, ironically, are easier to get around because when you define precise rules, they’re easier to skirt.
Instead, the AI Act recommends principles that act like an invisible hand to guide firms toward the ethical, agile use of AI and data. It’s principle-centric, not punitive-centric, which could accelerate its implementation.
The risk pyramid
The EU AI Act defines risk categories matched to applications and systems (see below). For example, applications like the use of AI in spam filters present little ethical risk, so they live at the “Minimal Risk” base of the pyramid, along with the majority of AI applications. These uses of AI carry no ethical obligation in the EU model–the more spam blocked, the better.
“Unacceptable Risk” applications of AI, on the other hand, are banned. Examples include the subliminal use of AI to influence decisions without disclosure, manipulation by embedding AI in toys without consent, or the use of facial recognition technology (FRT) for law enforcement.
Facial recognition is a good example of the AI ethics challenge. The technology is becoming easier to use, cheaper, and better able to identify the identity of people from long distances. But unlike many other forms of your personal data, your face can’t be encrypted. So unlike passwords, you can’t change your face. Data breaches involving FRT data must be avoided at all costs, and are banned.
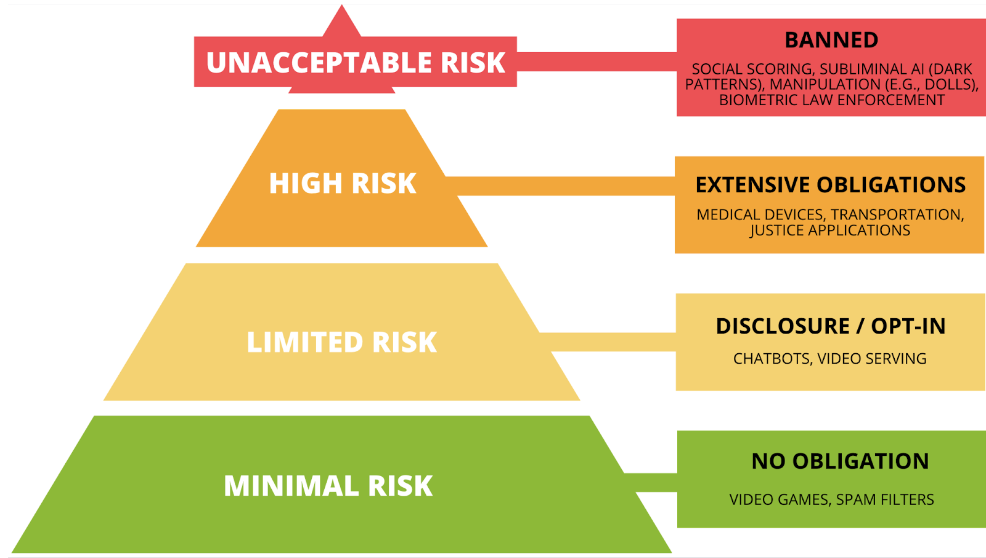
In the middle of the pyramid are where governance, risk management, and disclosure come into play. These applications tend to be used for good. Examples include the use of algorithms in medical devices, chatbots and video serving. This use of AI comes with the responsibility to disclose or govern algorithms.
To address this responsibility, the AI Act proposes seven principles that any data leader can use as guardrails to vet and scale policies:
- Human agency and oversight
- Technical robustness and safety
- Privacy and data governance
- Transparency
- Diversity, non-discrimination, fairness
- Societal and environmental well-being
- Accountability
These principles are covered in depth from a data scientist’s point of view, along with some detailed ideas on how to implement them, in Seven Steps to Reduce AI Bias.
The role of data in the AI EU Act
Data is addressed in the AI EU Act in principle three, which says that the data used by AI should adhere to privacy and governance principles. While that’s a good start, data should have a more prominent role in future regulation because without data, AI does nothing. The two are inexorably linked.
That said, the EU AI Act fills the void of AI ethics conversations today. Data is in the conversation, and even in its current form, it’s sparking change and debate.
Making the AI Act your own
If you’re a data leader, it’s time to explore the AI Wild West so you can begin to get a sense for the ethics of AI. Here are five first steps:
- Understand how, why, and where data is being used to power AI. It’s no longer acceptable to “assume it’s someone else’s job” to vet how algorithms use data. Do you check at all? Do you track the relationship between algorithm training data and operational scoring data? Start by taking inventory of where data is used by algorithms and how it’s used in the life cycle of AI. A good place to start is to read Chapter One of 10 Things to Know About ModelOps.
- Embrace data scientists. Data scientists usually aren’t computer scientists – they’re focused on math more than software quality, performance, testing, scale and reliability. Engage them to understand what data they’re using and how their models are used by the business. Work to educate them about the ethical and compliance implications of the data they choose and use.
- Promote and embrace Decision Observers. AI brings new challenges for CDOs, because algorithms generate predictions, and predictions are a new, synthetic source of information. Engage teams to understand synthetic data, how it should be managed, and how it's used in decision-making.
- Evangelize this: AI is a team sport. The CDO is the straw that stirs the enterprise data drink. With AI, data leaders must engage data scientists, Decision Observers, analysts and business leaders. More than most other uses of data, AI is a team sport.
- Get started. Read the full EU AI Act and start to apply its principles to your organization. It’s no longer acceptable to pass data along and hope it’s used properly. The Act lays out principles to engage data science teams, identify and mitigate risk, and creates a cultural framework that will help open a dialogue with your team.
As you’re breaking down your enterprise data stovepipes, kick down the mathematical ones, too. Like human bias, you may have blind spots. It’s early, and that’s ok. But remember, there’s a reason all that money is being invested in AI technology. It’s becoming an increasingly dominant matter of business success and, indeed, survival. Everyone is watching and playing the AI game. Join in