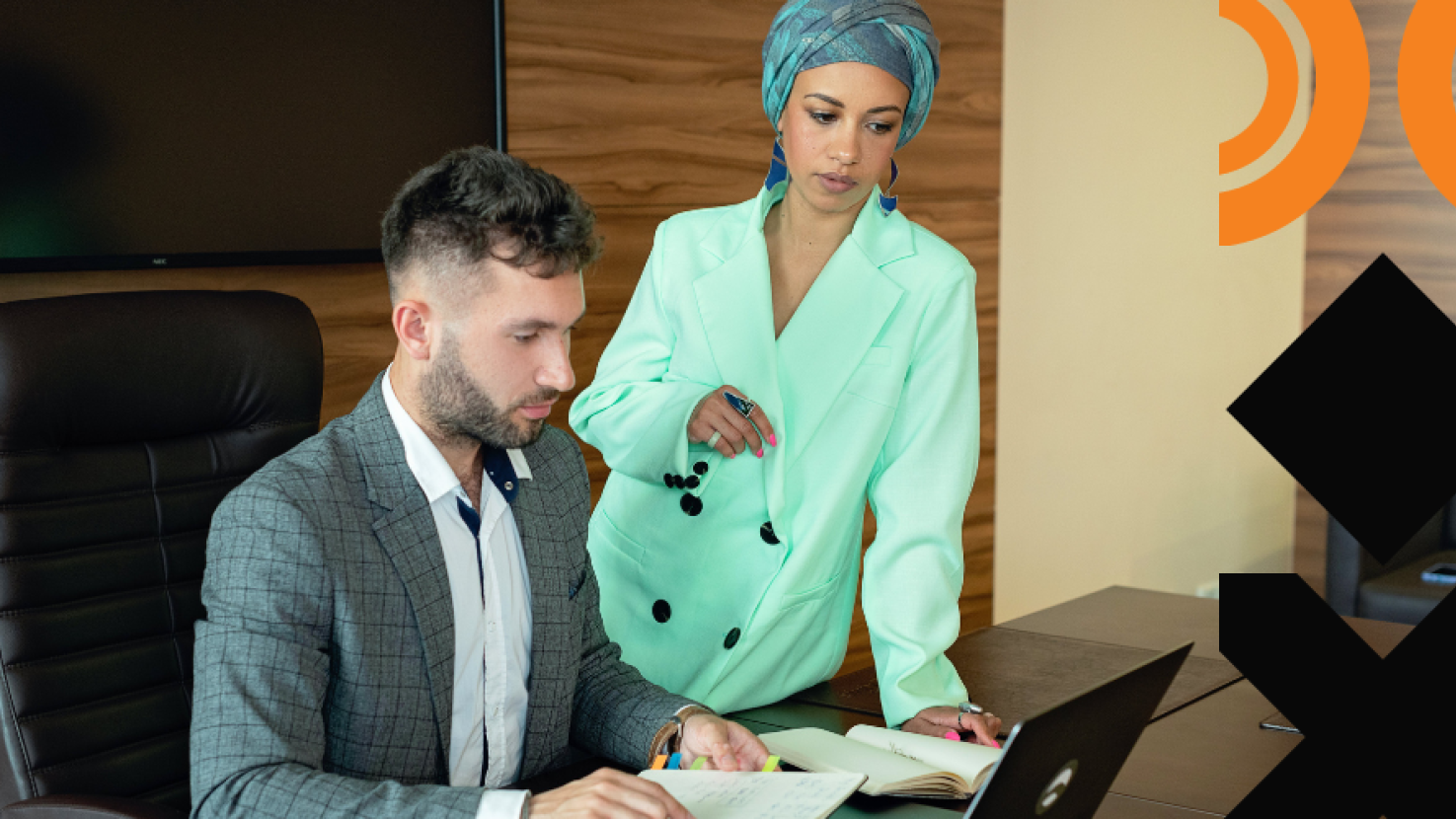

AI and Data Ethics: What a Data Leader Needs to Know
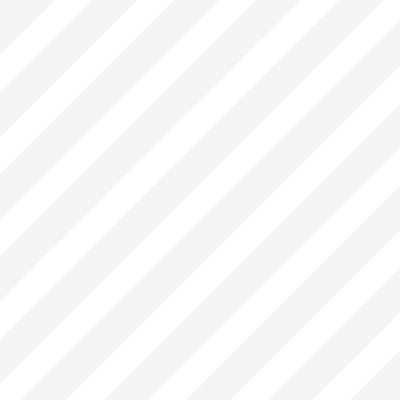
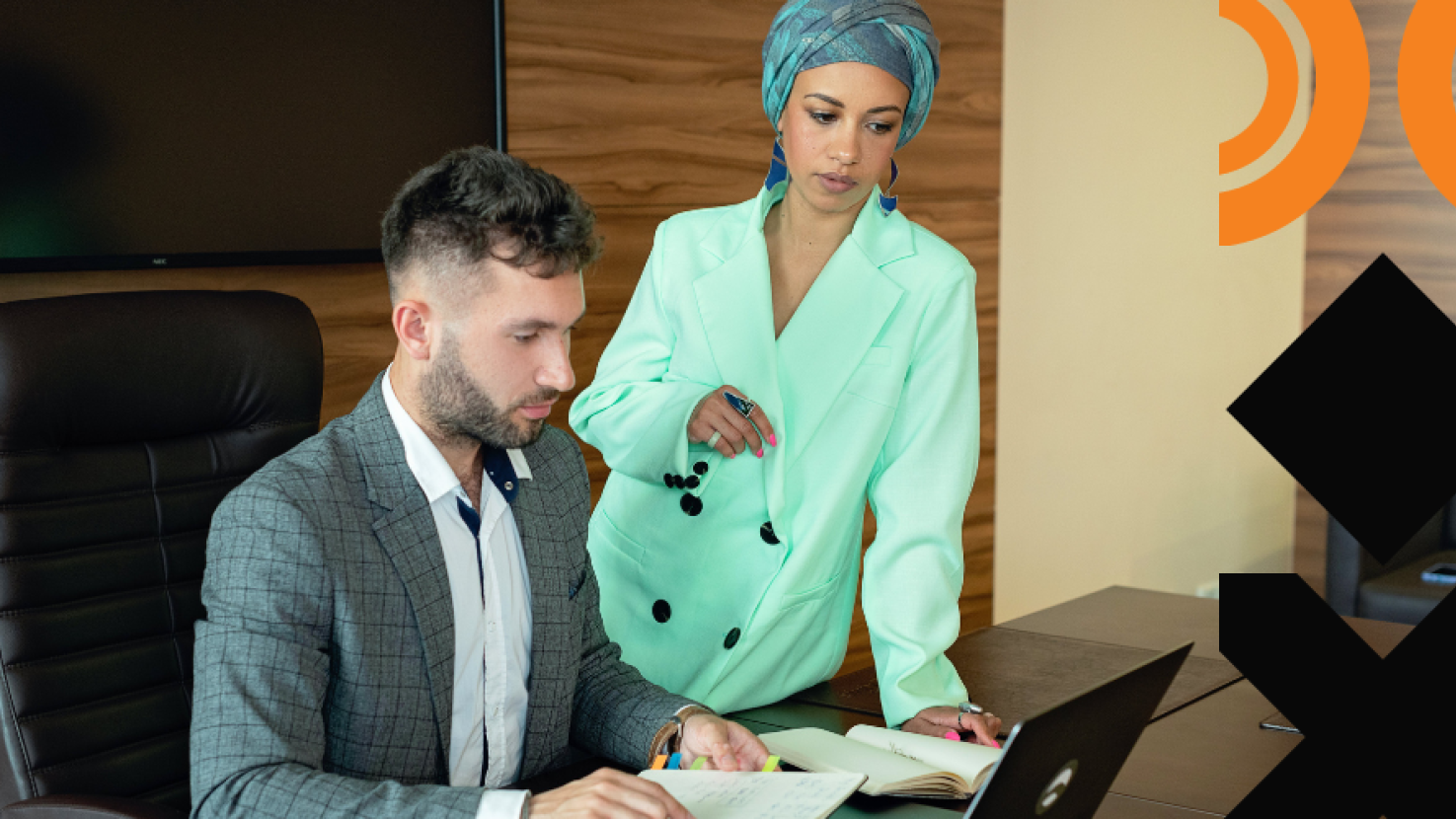
Organizations of all types are trying to make sense of AI and what it means for them. I put its potential on the order of the discovery of electricity and believe it is too soon to assess its long-term impact, even if some business analysts call it overhyped today. However, the impacts of AI may not be obvious since AI is being misrepresented and misperceived, as computational innovations always have, dating back to the 1950s. GenAI based on large language models (LLMs) is unlikely to meet science fiction-tinged expectations that it will accomplish any intellectual task we give it, but is likely to have significant impact where its effects are less visible and less widely perceived.
One area to pay attention to is the effect AI will have on replacing work currently performed by cognitive professionals, such as those in medicine, law, engineering, etc. The effect here will be twofold – the disruption of labor and the impact of recipients of the services provided by these professions. This is why the ethics of AI is a subject that we can’t overlook.
The problem of bias is exponentially more important in AI models because LLMs are much more dependent upon the data they are trained than other kinds of models. They are all based on a very sophisticated form of what linguists call the distributional hypothesis. Simply stated, for all the claims that it is an “intelligence,” GenAI is just models looking at probabilistic distributions of words and making plausible sentences based on billions of sentences that have previously been uttered. That’s why the makers of GenAI models have a hard time overcoming the biases that are in its original dataset. That is one of the biggest challenges with AI and ethics — the bias is not intentional, in the same way that any specific culture is merely the accumulation of adaptive biases over time. What we’re looking to root out is harmful bias, anything that might lead to a negative evaluation on the basis of characteristics like gender, race, physical ability, zip code, and so on.
Fine-tune. Curate. Repeat.
Because of GenAI’s dependence on data, it behooves us to be careful about the collection and curation of the data that goes into the models. Currently, many projects are training and fine-tuning specialized data models based on well-defined data sets to ensure that harmful bias is minimized and validity maximized. A data model trained on PubMed articles is unlikely to make the same kinds of errors that a less curated data model would when sharing information about medicine.
This curation trend is important because early LLMs have been trained on an indiscriminate mix of content that’s as likely to be from a pornographic site as it is from a reference work. Negatively biased correlations between images and language will therefore be present, in both explicit and subtle forms, that might undermine your bottom line in domains relative to your business.
With this in mind, it’s important for data leaders to:
- Educate yourself on systematic biases or patterns that might be in an LLM you plan to use. This entails focusing on the first part of the data pipeline and the sources that went into the data model
- Be intentional about curation, the selection and the organization of data sources in your ultimate model
- Remember that big data cannot trump bad models, and that quality of data always should be a primary focus as industry moves to curating and developing smaller data sets to inform its activities
Data ethics and AI: incompatible?
AI technologists and data ethics practitioners may not see eye-to-eye today, but they must meet on common ground as quickly as possible. I would consider it a failure of the imagination if we cannot develop an ethical framework we can use to conceptualize the development of AI.
The ethics of AI is a social, regulatory, policy-based, “best minds thinking” kind of problem that we can focus on. It’s not an issue solved by binary thinking of an “it’s too vital to be regulated” camp versus an “it needs to be shut down right now” camp. We need to take a more nuanced view than just assuming that AI is an inherently dangerous thing or a miracle for the world if we want to properly address the ethical challenges that come with it.
In my view, the proper ethical perspectives for AI will come from two places in collaboration:
- AI-producing communities and developers such as Stanford’s Human-Centered Artificial Intelligence group and U Cal Berkeley’s Center for Human-Compatible AI. Both are developing frameworks called humanistic artificial intelligence, or AI that is built with humanistic values and concerns from the start. The value of groups like these is that they often think of ethical problems as machine learning problems that just need to be solved and built into models.
- Critical studies scholars from academia who argue that the solution is less about how you make a specific AI less biased or less harmful, and more about identifying the discourse of the questions that are framing these issues in the first place. The idea of privacy may sound like a good one, for instance, but today privacy is almost impossible to achieve. How then does privacy become woven into an AI model? As this second group develops findings, there must be dialogue between the two about implementing them.
- In addition, for AI to become truly ethical, data leaders need to lean forward, weigh in, and enter the conversation.
Parting thought
Finally, it is important to remember that an AI’s connection to truth is indirect, even if its ability to produce coherent and often useful sentences is strong. GenAIs have no notion of truth, one way or another. A growth area will be in the connecting these models to agents that interact with the external world where their “ideas” can be subject to experience, failure, and revision, beyond the current reinforcement learning methods currently being employed.