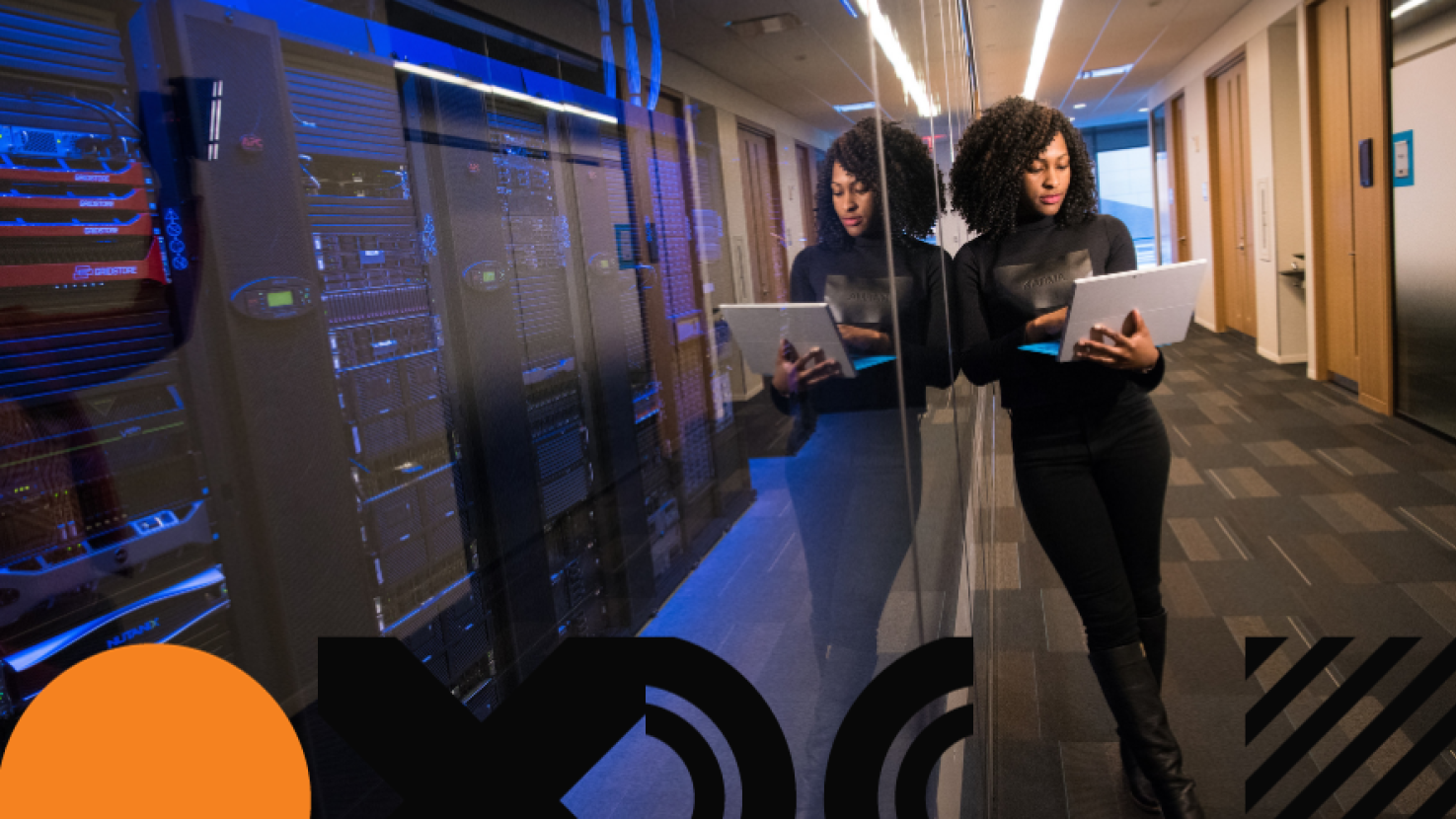

5 Ethical Principles of Data Collection
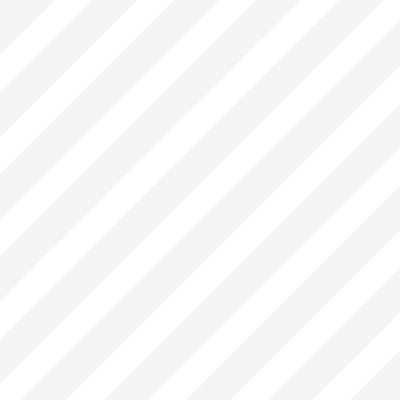
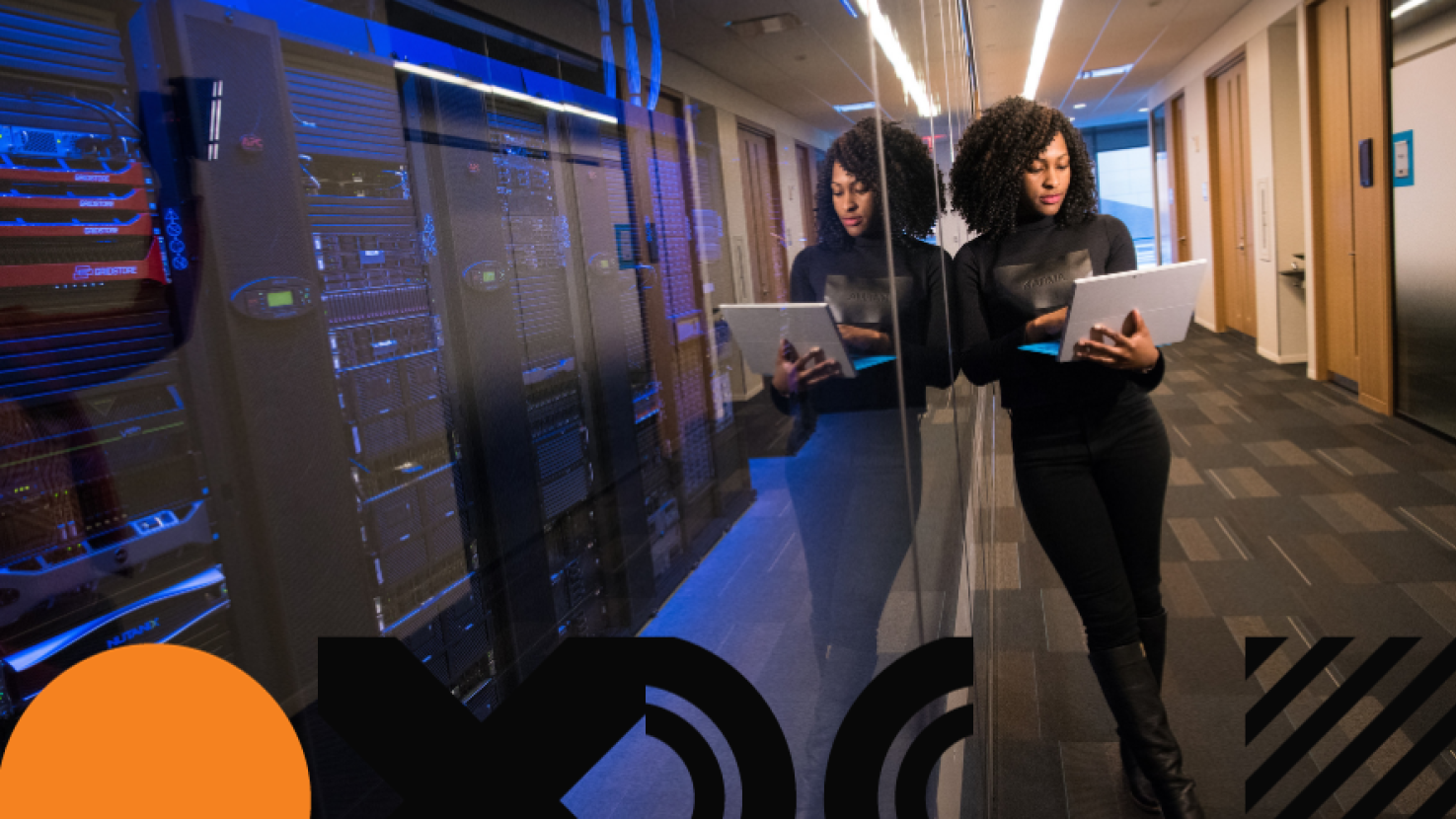
Whether I’m talking to students, developers, or clients, I like to frame data ethics as a real-world, in-the-moment activity. If you sit between raw data and how that data is presented, you’re not just clicking the buttons and writing code – you’re a narrative maker. The onus is on you to think through what you’re putting out there – which data points to show, how to communicate them, how much the user can manipulate them, which filters to use – whether you’re doing it yourself or on behalf of a colleague or employer.
It's important to stress all of this because people aren’t as aware of the ethics of data as they should be. As a result, they’re much more likely to do what a computer is telling them rather than to push back against it. Nearly everyone is likely to say, “Well, the computer says X” as if this machine that we’ve programmed is an arbiter of truth.
Here are some of the ethical principles in data collection I find valuable, and that data leaders can use to help people take a step back and reassess their ethical role in the data they collect and present.
- You do, in fact, have control. As a data culture, we’ve become too accustomed to presentation tools doing the decision-making for us. In fact, we have control over the data in a dashboard as much as we do the charts, filters and fonts we select. It’s critical that we don’t allow our teams to abdicate responsibility here, and we have to be just as present and intentional about these decisions ourselves.
- What you do with data has real implications. Genetic information services like 23andMe or Ancestry.com are presented as engines of personal liberation and insight with the personal data they provide. Yet what if you’re an adopted child, reach out to relatives you never knew you had, and discover that they have no interest in knowing about you? Or what if you’re tasked to present infection rate data to the public, and you prefer to divide the number of infected people against the total sample whereas your employer wants you to divide by the total number of tests taken instead? It’s important to think through what you do with data, and how you share it, before taking action.
- Demand transparency. When presented with data, ask yourself or the team involved some basic questions
- Why was this data collected?
- Who did it come from?
- Who was collecting it?
- What’s the nature of the data sample?
- Who or what was the data sample trained on?
These are very common sense questions, but it’s surprising how rarely they’re asked. Encourage your team to ladder down with questions the same way a three-year-old does. There is almost always another “Why?” question that can surface data intent.
- In an age of AI, look for explainability. There’s a reason many public firms have banned the use of ChatGPT at work, and that’s explainability. Data cobbled together from many sources and presented based on similarity algorithms can be rife with hallucinations and errors. If the data you’re analyzing is a black box, where does accountability lie? How do you know what was done to the data, and do you agree with it? Accepting data without question is giving your power over to the entity that is presenting it to you.
- Is the data accurate? Accuracy, precision, and completeness are key measures of data quality in any circumstances, but are particularly important when viewing data through an ethical lens. If the data is of low or suspect quality, the results and insights will be as well, and this can have serious societal consequences.
Conversations about data ethics tend to start and end with privacy and personally identifiable information. In my academic work I like push students to go deeper and constantly question their actions and the ethical implications of the data they consume and use. For example:
- Where’s the data coming from?
- What were the methods used to collect it?
- What do we value or not value in the outcomes?
- If a mistake was made, who’s accountable?
- What are we going to do with this information?
- What does it really matter to us?
- Are there issues here with different truths?
- What’s the rationale for the way we want to calculate something?
Questions like these are usefully adjacent to data governance and master data management, which define large parts of practical data literacy. That would lead me to a final recommendation about data ethics. Don’t think about it as a standalone topic, the session at a coding conference that’s going to draw the lowest number of participants because people think of it a bit of a bore.
Instead, encourage your team to dig at these issues around the ethical principles of data collection in context. When you start exploring the implications of analyzing COVID data one way versus another, what happens when an AI healthcare application limits its data corpus to wealthy people, or the output of a hiring bot that optimizes its decision set by prioritizing men, your colleagues will start to see the people underneath all these decisions about data. In this way we’re all united, and the ethics of data should speak to all of us.