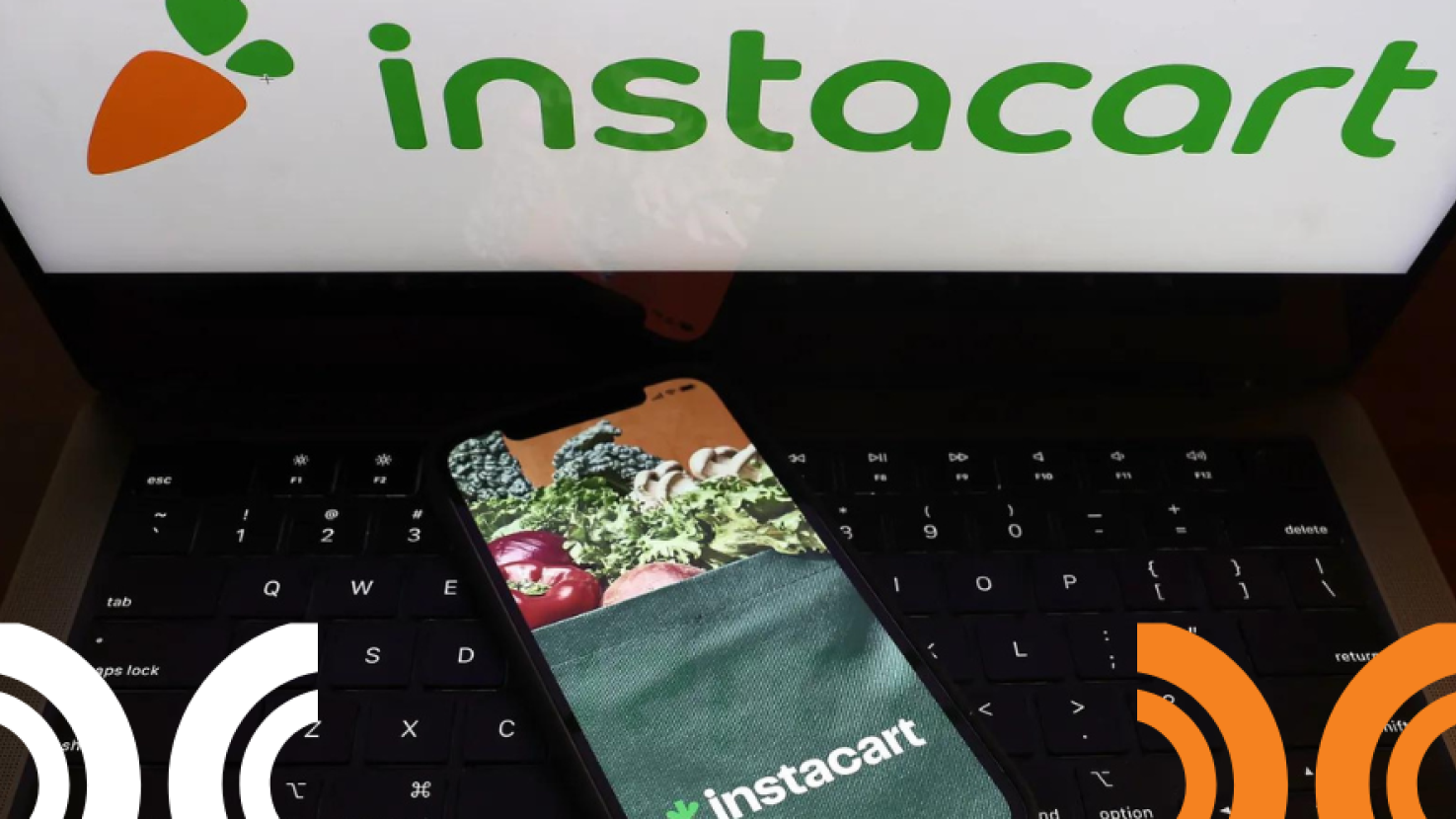

Anahita Tafvizi: A Data Leader Pioneering Innovation at Instacart
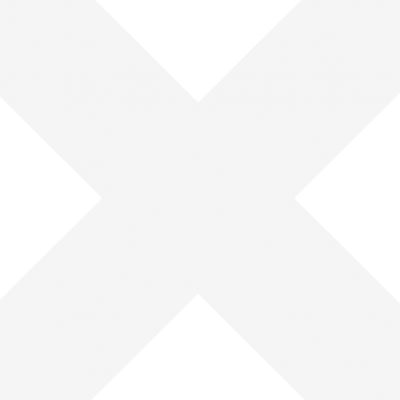
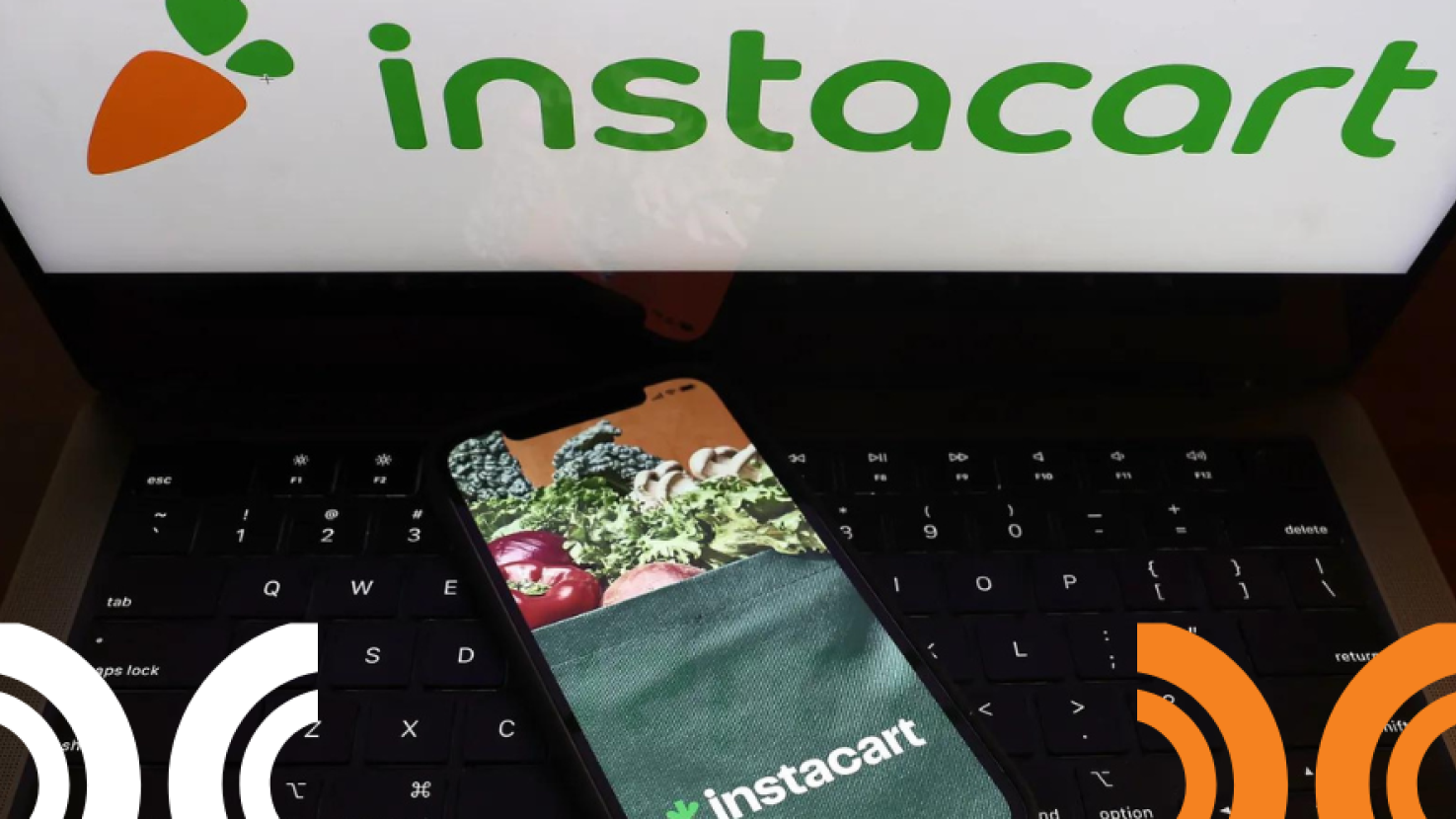
Anahita Tafvizi is no stranger to data-intensive industries. From her early career as a physics researcher at Harvard and MIT to holding top roles in data science, finance and strategy and operations at eBay, YouTube, and Google, she has honed her expertise in machine learning, predictive analytics, and data-driven product strategy. In her current role as Vice President and head of Data & Analytics at Instacart, she leads the enterprise’s data science and analytics strategy, overseeing a team of more than 150 data professionals. Data Leadership Collaborative recently spoke with Anahita to explore how Instacart maximizes its data and to get her insights on the challenges many data leaders face today.
DLC: How can the retail and grocery industries benefit from predictive analytics, and how is Instacart leveraging these capabilities in the e-commerce space?
Anahita Tafvizi: Retail is one of the sectors that can be profoundly data-driven, and predictive analytics is pivotal for driving enterprise strategy and financial success. Traditional retail questions—such as pricing, inventory analysis, store aisle management, and demand forecasting—are significantly enhanced through the application of data and machine-learning techniques. By analyzing historical sales trends, understanding the interdependencies among product sales, and monitoring competitor pricing, we can forecast inventory needs with greater accuracy.
In the e-commerce space, these principles take on new dimensions. For instance, aisle configuration in a physical store translates into page ranking in an online environment. At Instacart, we leverage our extensive consumer data to personalize shopping experiences over time. Similarly, store promotions are mirrored in our online advertising strategies. The critical trade-offs between growth and profitability, as well as pricing and inventory forecasting, are just as crucial—if not more so—in e-commerce. The richer access to data in the e-commerce realm further amplifies the value of data-driven optimization and machine learning.
We also employ data-driven approaches to inform product decisions. For every launch, we conduct experiments using state-of-the-art techniques, allowing us to make more precise and timely decisions. For example, by using predictive modeling based on past purchases at the user level, we enhanced our experimentation velocity through variance reduction.
Another key area where predictive analytics plays a vital role is in driving growth and optimizing marketing strategies. For example, habituation - encouraging customers to form shopping habits - is crucial for sustained growth. Through predictive modeling, we identified key behaviors correlated with customers building sustained shopping habits and then developed, implemented, and measured a product and marketing roadmap to shape these behaviors. We then further validated causality through rigorous experimentation.
DLC: Some tech companies prioritize their initiatives through sales feedback, while others rely on product and user research. Would you consider Instacart to be product-led or sales-led?
Anahita Tafvizi: I would describe Instacart as data-led, though I might be a bit biased in saying that. Our data-driven decision-making is not just a theoretical framework; it’s a practical reality embedded in everything we do.
We follow a structured approach: understand, identify, execute, and measure. Data shapes our understanding and priorities and enables us to assess the accuracy of our hypotheses. A significant credit goes to our CEO and our leadership, who have championed our transformation into a truly data-driven organization. Their support has empowered us to integrate data at every level, making us proactive in deriving insights that drive business decisions.
Our centralized data science team plays a crucial role in identifying and sizing opportunities, ensuring we prioritize initiatives with the greatest potential impact. We leverage an extensive array of causal inference techniques, including scaling randomized A/B tests across the entire company, to evaluate impact and understand trade-offs. This approach is integral to our planning process, providing the data that underpins our strategic decisions. We also conduct weekly business reviews with the leadership team to assess execution progress and share the latest insights, contributing to continuous iteration and refinement.
DLC: Generative AI has come a long way in just over a year, but many companies are still in the midst of small-scale experiments and proofs of concept. How is Instacart leveraging Generative AI?
Anahita Tafvizi: At Instacart, we leverage Generative AI in two key areas: consumer-facing products and internal operations.
On the product side, it’s fascinating to see how we can better support our customers as Generative AI evolves. We provide real-time support through Carebot, Instacart’s LLM-powered customer care chatbot, which responds to requests around the clock and can take action on behalf of the customer. Additionally, we leverage Generative AI throughout the grocery basket-building journey via our AI-powered search tool, Ask Instacart. This tool can answer questions like “Can you recommend a gluten-free snack?” and supports hyper-personalization of storefronts and AI-generated content to better meet user needs.
Internally, we developed a tool called Ava, which leverages models from OpenAI and other leading providers, combined with Instacart’s proprietary data, to handle tasks ranging from document summarization and interview notes to code generation and labeling unstructured data for analysis. These code-writing co-pilots have been so successful that they’ve transformed our interview process for the data science team. We no longer ask candidates to write SQL queries from scratch; instead, we focus on prompts to generate SQL queries and methods to verify their accuracy.
We’ve also harnessed LLMs to improve our data pipelines, making them more efficient, faster, and cost-effective. As data scientists, embracing LLMs has been a priority, allowing us to accelerate our workflows and enhance accuracy.
DLC: You have a significant remit in your current role. How do you approach the training and talent development aspects of your data team?
Anahita Tafvizi: Growing and investing in the talent within my team has always been a top priority. I focus on cultivating a performance culture that motivates and challenges top performers. As a leader, it’s essential to identify and invest in your highest-potential talent, which not only helps retain them but also supports their long-term career growth.
Providing consistent, real-time feedback—both positive and constructive—is another critical element of my approach. Direct feedback allows individuals to quickly adapt and continuously learn. I also strive to be decisive and follow through on my commitments, both within my team and with external partners, to build trust and ensure alignment.
We recently launched a program within my organization called “Foundation Fridays,” a monthly initiative that includes structured programming, such as training sessions and talks from external speakers, followed by dedicated deep work time. This initiative allows team members to hone their craft, improve workflows, practice new skill sets, and engage in peer mentoring. During these sessions, we emphasize the development of technical skills, including investing in our data pipelines, expanding our technical toolkits, and exploring new quantitative methods. I believe this focused time investment is crucial for keeping the team at the forefront of innovation and fostering a culture of continuous learning and knowledge sharing
DLC: How should a data leader approach thought leadership in data analytics as a way of establishing an influential voice in the industry?
Anahita Tafvizi: Thought leadership in data analytics is a strategic asset for building credibility and influence within the industry. By sharing insights, best practices, and innovative strategies, data leaders can demonstrate their expertise and establish themselves as forward-thinking voices. This can be achieved through active participation in industry conferences, publishing articles and whitepapers, and engaging in discussions on platforms like LinkedIn.
In addition to elevating their organization, thought leaders have the opportunity to influence industry standards, regulations, and best practices. By taking on advisory roles or collaborating with academic institutions, they can help shape the future of data science education, ensuring that the next generation of professionals is well-prepared to meet the evolving demands of the field.
Data leaders should also consider the ethical implications of their work. As advocates for responsible AI and data practices, they can shape conversations around critical issues like data privacy, bias, and the ethical use of analytics.
Finally, thought leadership is essential in mentoring the next generation of data professionals and building a strong community within the field. Through formal mentorship programs, hosting webinars, or producing content that addresses common challenges, data leaders can inspire and guide others, thereby enhancing their organization’s competitive edge and advancing the field of data analytics.