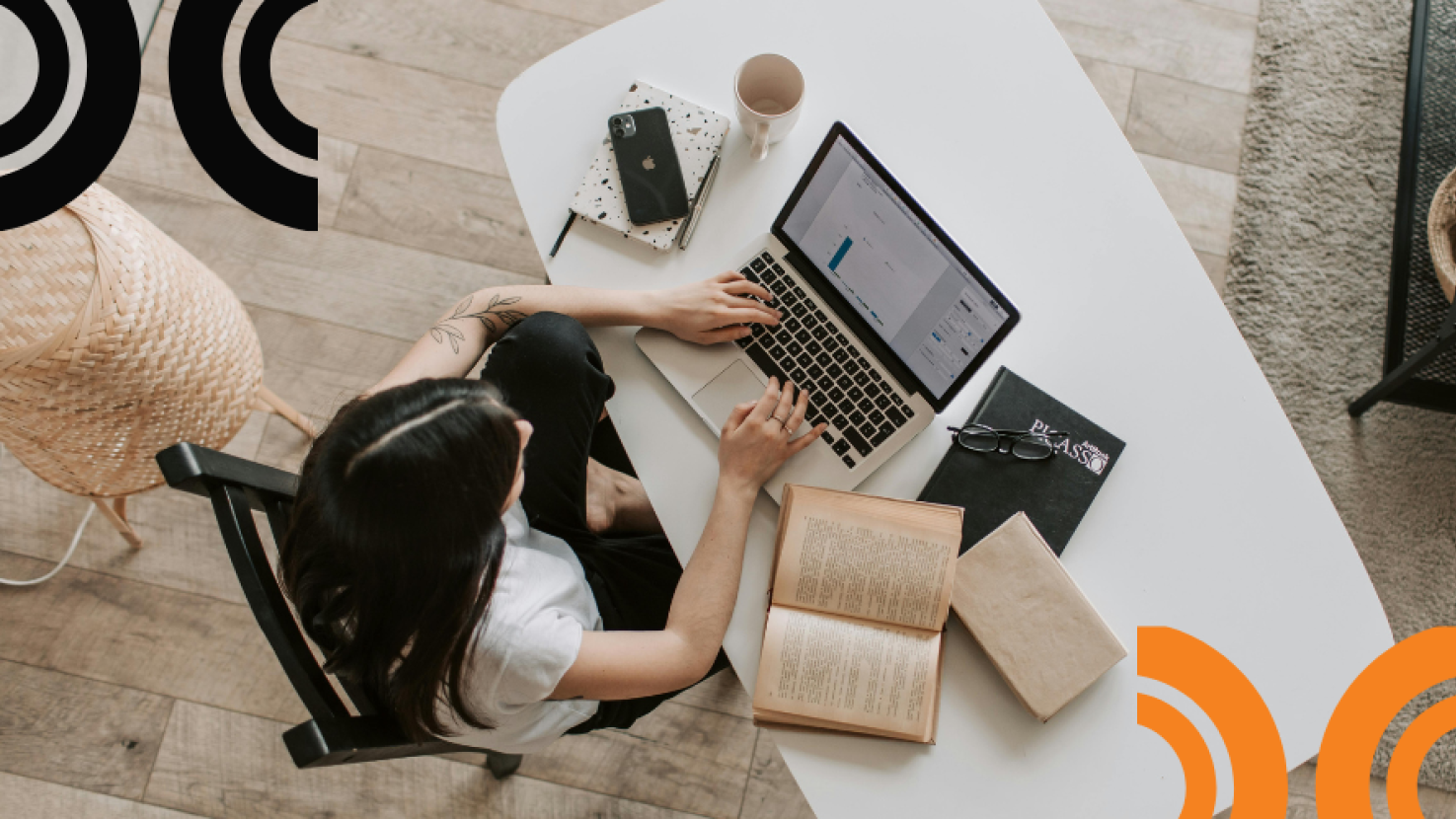

5 Things that Must Be in Place Before Launching Any Large-Scale AI Project
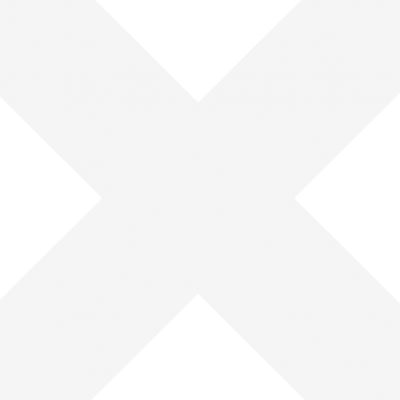
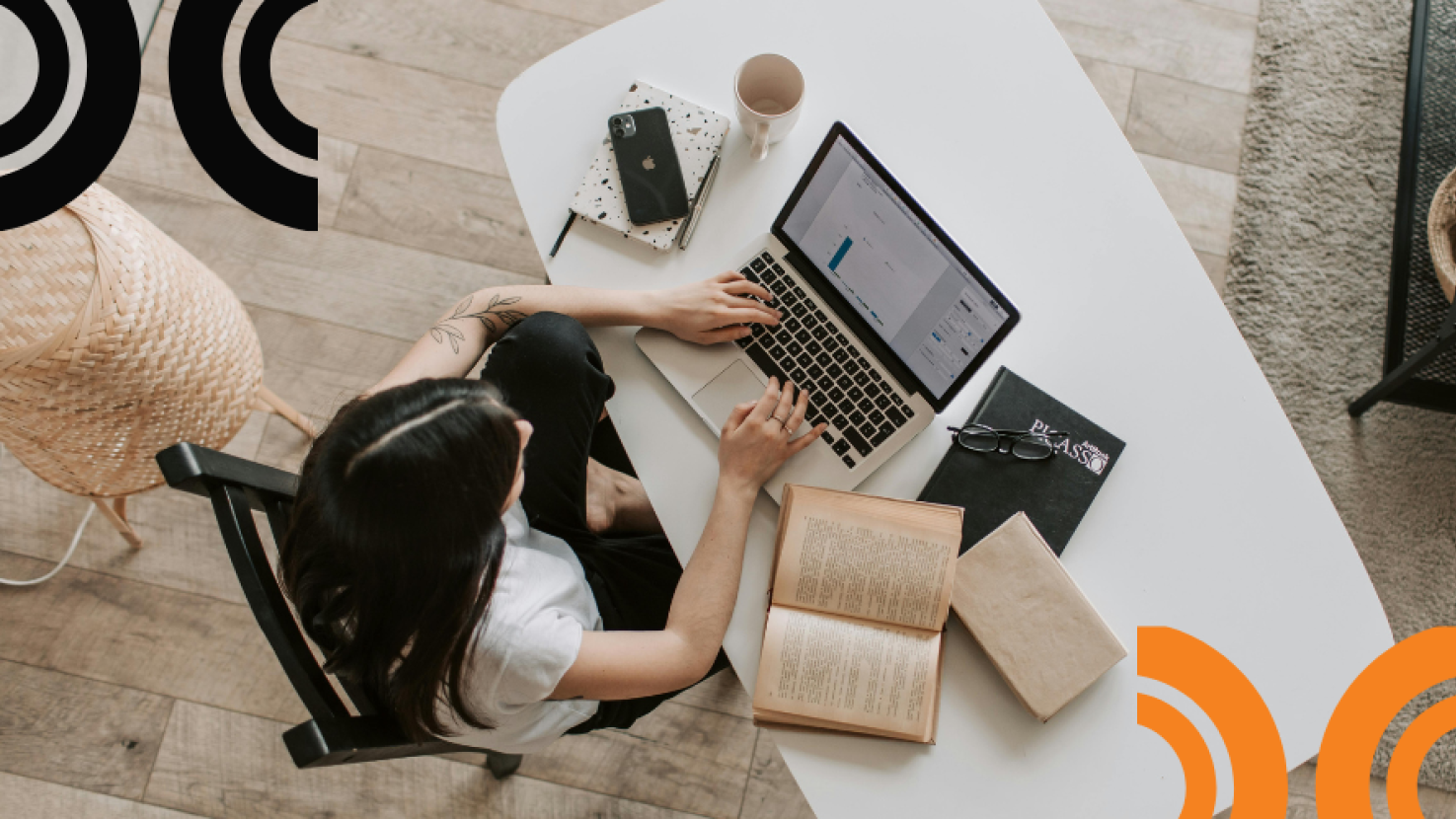
The past year in AI was about a lot of pilot programs, proofs of concept, and some real projects. At the very least, data leaders collected a lot of learnings. For example, we learned that:
- AI projects are iterative by design
- Each project needs a wealth of data to get moving
- It’s not just about AI technology, but about interacting with existing systems and processes
Consider a new AI project such as building an AI chatbot for a retail sector client in the automotive space. It’s likely to have a few false starts, perhaps showing a customer non-hybrid models when they asked to see only hybrids or EVs. These false steps might be based on insufficient data or integration with existing systems. But thanks to iterative input from users and IT testing of new versions, the chatbot will be enhanced to handle more complex interactions and trained to get to better responses more accurately.
Scaling your new AI project
Now imagine scaling up this simple chatbot to an AI application that spans several lines of business, or an entire enterprise. Here are several things you should consider having on hand as you start to scale such an AI project.
- A clear objective. A crystal clear objective is crucial. In 2023 people just wanted to get into GenAI. But a desire for the new shiny object is not a business objective. What is it you need to do? Are you looking optimize your supply chain logistics, to reduce delivery times, to lower costs? Drill into the specifics with your team, because you’ll need those details when you get to step two.
- Executive sponsorship and stakeholder buy-in. Strong executive sponsorship is vital because large-scale AI projects require both significant resources and cross-departmental collaboration. By sponsorship I mean more than checking a box. This is about identifying someone who will champion your goals from plan to prototype to finish – followed by ensuring that all stakeholders are on board and understand the project’s goals and potential impacts.
- Robust data infrastructure. By now companies have figured out that they can’t embark on any AI project until they have sorted their data infrastructure. Perhaps more so than in any other realm of data and analytics, in AI, garbage in equals garbage out. AI models are only as good as the data they are trained on. So, it’s critical to have clean, well-organized and bias-free data. Moreover, if your data is siloed in different systems across various regions, unstructured as well as structured, labeled as well as unlabeled, you may be looking at six months to a year of formatting and integration work before you start on the new AI project proper. A strong data governance practice is key to manage data accessibility, quality and security.
- Scalable technology and tools. When considering the tech backbone needed for your AI project, the focus must be on selecting a tech stack that not only meets your current needs but also can scale effectively as demands grow. In AI especially, computational demands can escalate rapidly. Also, if you get the foundation right, you can build something substantial on top of it. Success is about ensuring these systems can communicate seamlessly with existing infrastructures and have the capacity to handle volume, velocity, and variety of data.
- A complete team. A group of dedicated AI and data science experts supported by a project manager and a few domain experts is enough to get going but insufficient for success. What’s left out of this team? Change management specialists. If you don’t have people who understand both the business and the technology side and can help user groups to transition to using your new AI products across the company, all your hard work may stall out. Culture change is one of the hardest thing that we do as data leaders, and if you aren’t equipped to handle change management, your project is likely to fail. Although it sounds counterintuitive, AI is all about people.
Where data leaders can help
With so much attention focused on AI today, the data leader must be prepared to play multiple roles in the organization. For example, they must
- bridge the technology-business gap that often exists with the C-suite
- have a robust data governance framework, because inconsistencies in how data is being handled that might have been manageable at small scale become a significant roadblock at large scale
- invest in advanced data management tools
- ensure that data is both available and of high quality
- have a realistic data integration strategy to merge data from different sources, formats, standards, and quality
- adhere to all data standards and regulations that are coming to market
- covering off on all facets of privacy, data bias, and ethics.
Data ethics and security and privacy issues are a particular passion of mine and of course go far beyond technical issues. They are fundamental to how we as a society are choosing to deploy technology responsibly. If we don’t manage them responsibly, things quickly can go to a dangerous area. Deepfakes are only one example. So as data leaders we have a very important job at our hands right now, to ensure that everyone from technical to legal experts are collaborating on how data is governed. I believe that trust will be the currency of future in the digital economy because there’s no dearth of content out there right now. But if your customers can trust you and your businesses, that is how you’re going to progress.
In the meantime, we’ve seen remarkable developments in AI. In nearly every organization GenAI already is improving the process by which content is being produced and managed. In marketing, these AI solutions are bringing measurable efficiency to creating and producing multiple versions of digital ads for companies. In retail, AI chatbots have taken a quantum leap forward in terms of their ability to understand nuance and complexity.
We do have a long way to go, but the scale of successful AI has made remarkable progress in less than two years, and that’s something to celebrate.