

Why Data Quality Should Be in Everyone’s Objectives
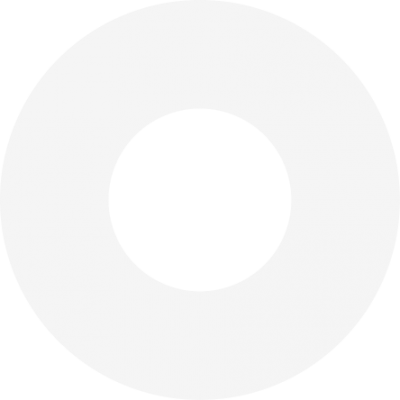

Last year, RBS International undertook three key approaches to boost data quality across the organisation:
- We added data quality improvement targets into everyone’s job goals.
- We instituted a company-wide Managing Our Performance (MOP) exercise, putting a spotlight on improving data quality.
- We introduced a new data quality framework consisting of six pillars, providing a holistic approach to tackle and improve data.
If you have a hard time imagining doing one of these things and developing data quality best practices in your organisation, it’s more achievable than you may think.
Data Quality Performance Goals
“Don’t walk by data issues” is part of the organisation’s data culture. Having active sponsorship from the CEO downwards is critical. Equally important is encouraging critical performance behaviours such as ‘critical thinking’ within performance goals. The following represents part of my own performance goals:
I’ll take responsibility to resolve a problem with data quality and don’t walk by seeking help from within my team, department and wider organisation resources to address the situation. Where needed, I’ll take action to escalate data quality issues as needed.
I’ll look for new ideas and/or innovative ways of doing things to improve data quality within my team, department and across the organisation.
Managing Our Performance (MOP) – Data Quality
We use the MOP as a way of engaging our team members and empowering them to solve problems or take advantage of opportunities.
Colleagues across the bank were encouraged to conduct a MOP taking no more than 20 minutes to generate and select the five most powerful actions that can be undertaken to improve data quality within 48 hours and not requiring additional resources.
Having the CEO, senior leadership team lead the way in sharing the results of their MOP cemented the importance of tackling data quality together.
Getting to know the root cause of data quality problems
When looking back at data quality related problems we faced over the last two years, we were able to group the problems into six areas which have formed the basis of our new data quality framework:
- Data Ownership
- Data Quality Design
- Data Quality Insights
- Data Quality Management
- Data Quality Improvement
- Data Quality Learning
When assessing how data quality is being addressed across the organisation or team, we typically start with data ownership and then data quality by design. Doing this first allows us to zero into problem areas very quickly, giving us a sense of how well critical and key data elements are being managed and improved.
You can imagine where these data elements might connect to existing programs. For example, if your business, like many, is trying to get a 360-degree view of customer insight across your products, channels, feedback, and any Net Promoter Score work, data quality management and data quality improvement can serve as important checkpoints and contributors to these efforts.
These efforts can then connect back into your business in the holistic picture you can offer relationship managers to help them have better informed discussions with the customers they're serving.
Conversely, if you’re experiencing any data trouble areas in your business, it’s likely that they will be tied to one of the parts of your framework. That may not make the problem easier to solve, but it certainly can narrow your search.
In addition to defining a framework, it’s valuable to create data quality insight dashboards to track and trace quality across the organization.
To stay aligned, meet regularly
Establishing, maintaining, and raising the quality of data does not happen on its own. I would recommend a regular data quality operational meeting. Aim for full representation across your organization. Invite healthy debates. Talk candidly about data quality and your culture of data but also about data integrity, data profiling and other data initiatives that are going on.
The good news is, these kinds of operational check-ins on data quality can catch on across your organization, and can be used to guide self-assessments. You can look at each franchise and function on, say, a monthly basis to ask, “What have you and your team done in the last month to move data quality forward?” Knowing that these questions will be coming creates a healthy sense of transparency, even if it’s sometimes heavy lifting.
Given the importance of data quality in almost every organization across nearly every industry, the question you might want to ask yourself is not “Why should I put data quality in everyone’s job goals?” but “Why haven’t I?”