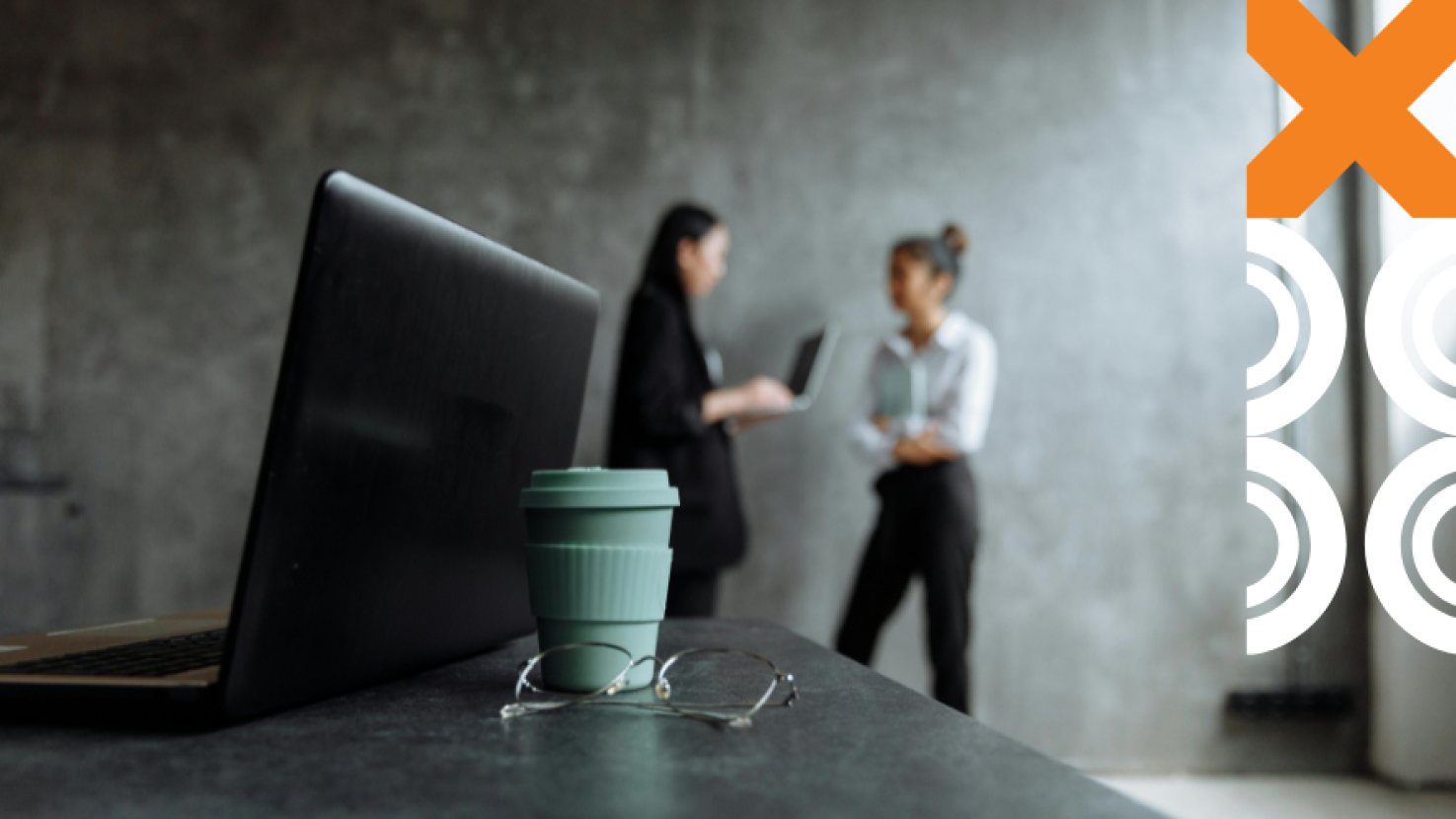

When Technically Right is not Correct
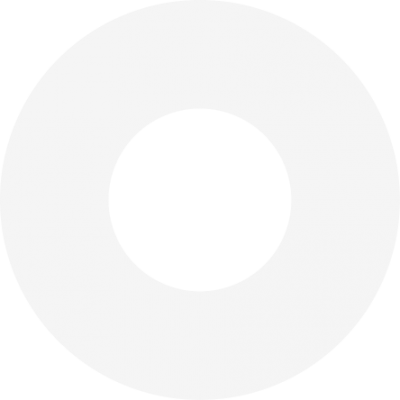
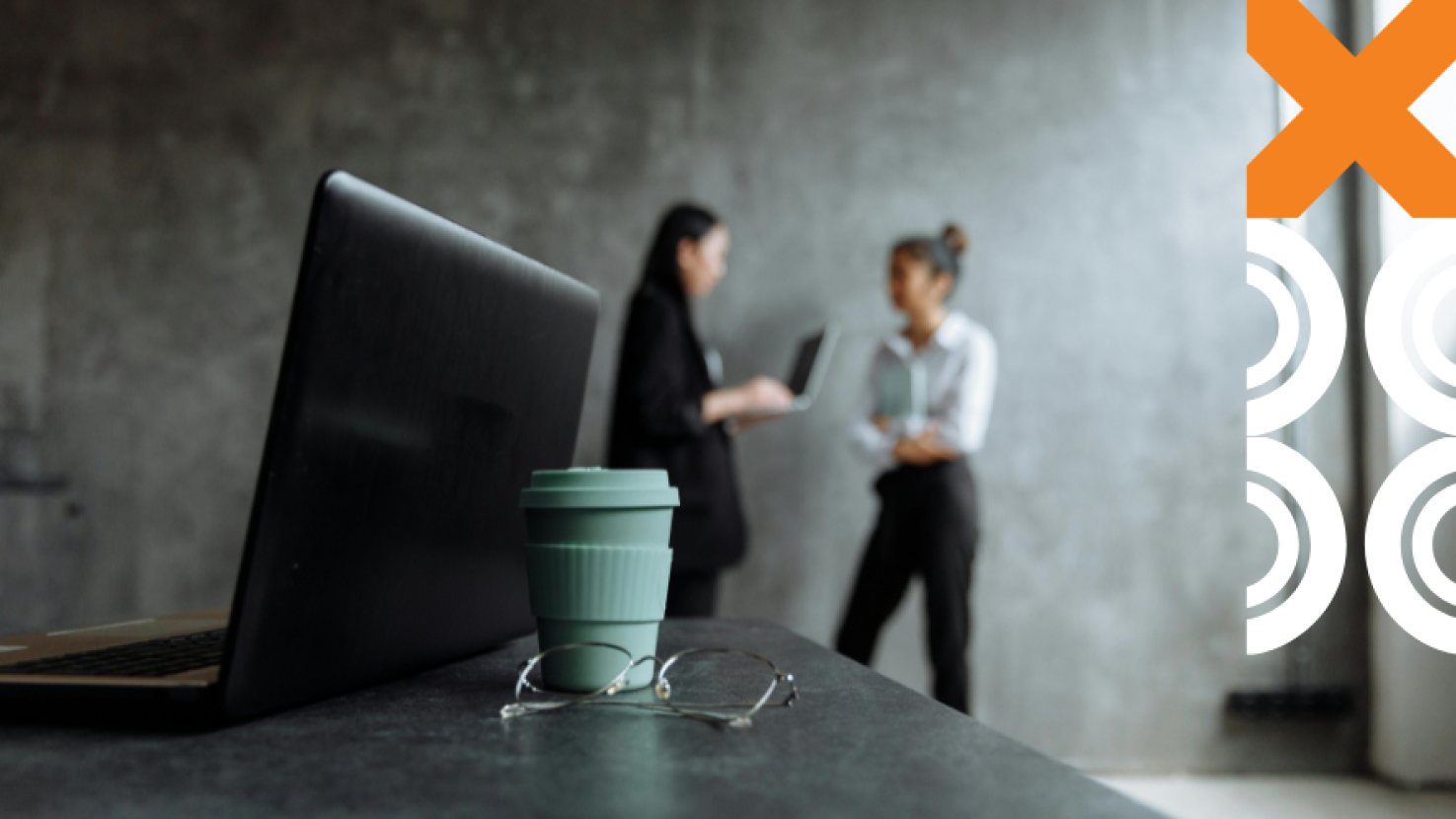
We all want to drive better decisions and create data-driven cultures, but how we achieve this goal will depend on our own skills and the skills of our teams. Many of us have, or rely on others with, high technical skills to solve complex business challenges. As data leaders, a common challenge we face is guiding these more technical staff to focus on business impact over executing challenging, technical work. It is up to you to balance and channel that passion without dampening those analysts’ spirits and motivation.
I have experienced this imbalance in the past, and I have made these mistakes myself.
Super smart (but super complex)
Early in my career, I worked on a strategy to inform a reorganization at a large commercial organization within my company. The decision-maker was an executive we will call Dan. I burned some midnight oil and came up with what I thought was a thorough and clever analysis. I had used some advanced statistics and built a fairly complex model to inform my recommendation, and I felt pretty proud of it.
One of my mentors at the time had a look at my work, took me aside, and said, “Colm, this is great, but Dan is not going to get this. He is going to be so distracted by the complexity that he will ignore the recommendations. I have no doubt that the logic is right, but this is not the framing you need to influence this audience.”
I was a bit disappointed, certainly, but I saw her point. If you are working with a CEO or other senior executive about a business issue, you should be able to preempt any hesitancy or anxiety around data literacy. If that does exist, you are not going to win their confidence coming back with a bunch of Greek letters. You are sending a message to this person that you do not understand the information context of your audience and that you have not done the work to translate technical complexity into simple, actionable insights. You will be far more successful by speaking the language your audience is comfortable with to build understanding and trust.
From data push to data pull
The greatest frustration I have seen from more technical colleagues who have worked for me has been situations where they feel like their work is not valued, and that they feel they have to sell the value of their work to the audience. You should always make your staff feel valued and appreciated, of course, but if they struggle to explain what an analysis is meant to tell the audience, there is a fault in the system somewhere.
As a data leader, you may need to do two things at this point:
- Create a shift from a push data model to a pull data model. This means that you and your analysts are directly engaging with your internal clients to understand where their focus is and what sort of data they need to make a decision. Once there is alignment between the two parties, you will begin to move past data literacy to what I call data hunger. You also evolve beyond the painful process of your analysts having to get people to pay attention.
- Make data analysts a more embedded part of business teams. Once a data analyst proves themselves capable of listening to a challenge and teasing out the level of data literacy in a specific organization, they are more likely to deliver something that hits the mark and creates a clearer path to sound decision-making. At that point, they will be invited back and ideally become an on-call resource, providing vital insight as well as a path to self-service. This is all part of the data literacy journey.
As a data leader, this is where you can play a role in supporting your teams. I think of it as bridging the literacy gap and working on the cultural norms to create a runway for the work to land successfully.
Remember that data literacy is a two-way street. You do not want to end up perpetually constrained by only being able to supply very basic insights; it is also your job to incrementally increase the data literacy in the organizations you work with, and develop the appetite for more complex work. You may have to start with the basics, however, build and iterate on your output, and go through that journey together with your stakeholders. If your team feels that their work is not effective, it may be that they have yet to build the rapport and understanding they need with their audience.
What I took away from my own experience continues to inform my perspective on creating successful data products:
- Know your audience.
- Focus on what you need to show to change perspectives.
- Take a decision-based perspective on your data output.
In my own case, I came back with a much clearer and simpler approach. It was all my executive audience needed.