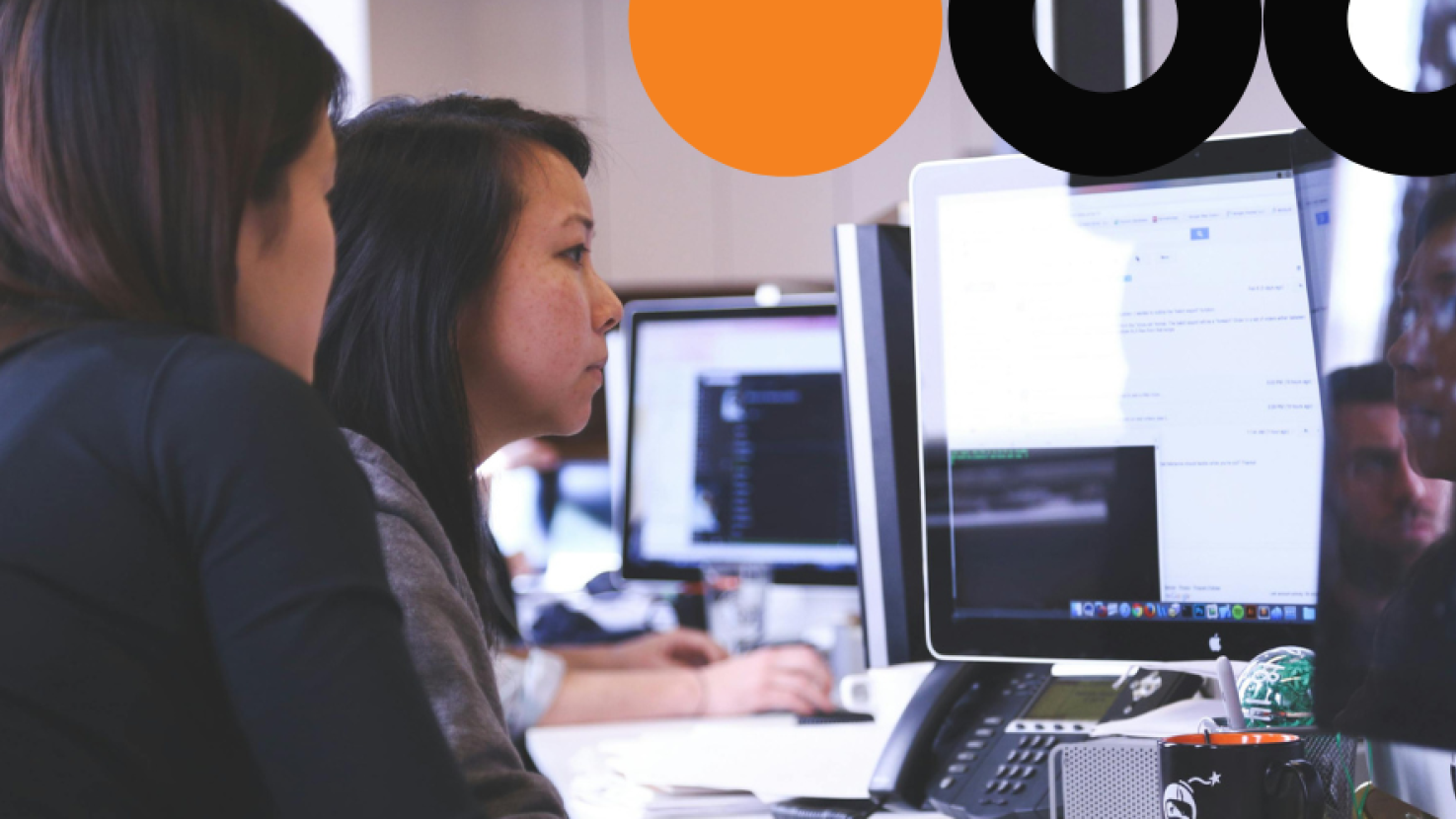

Five Ways to Build a Better Data Product
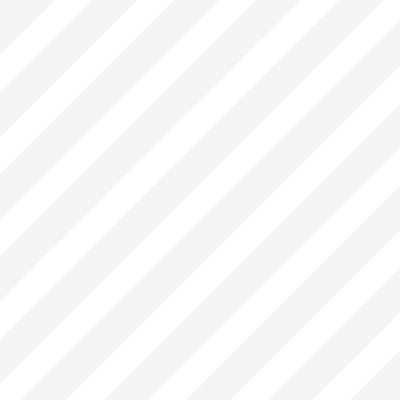
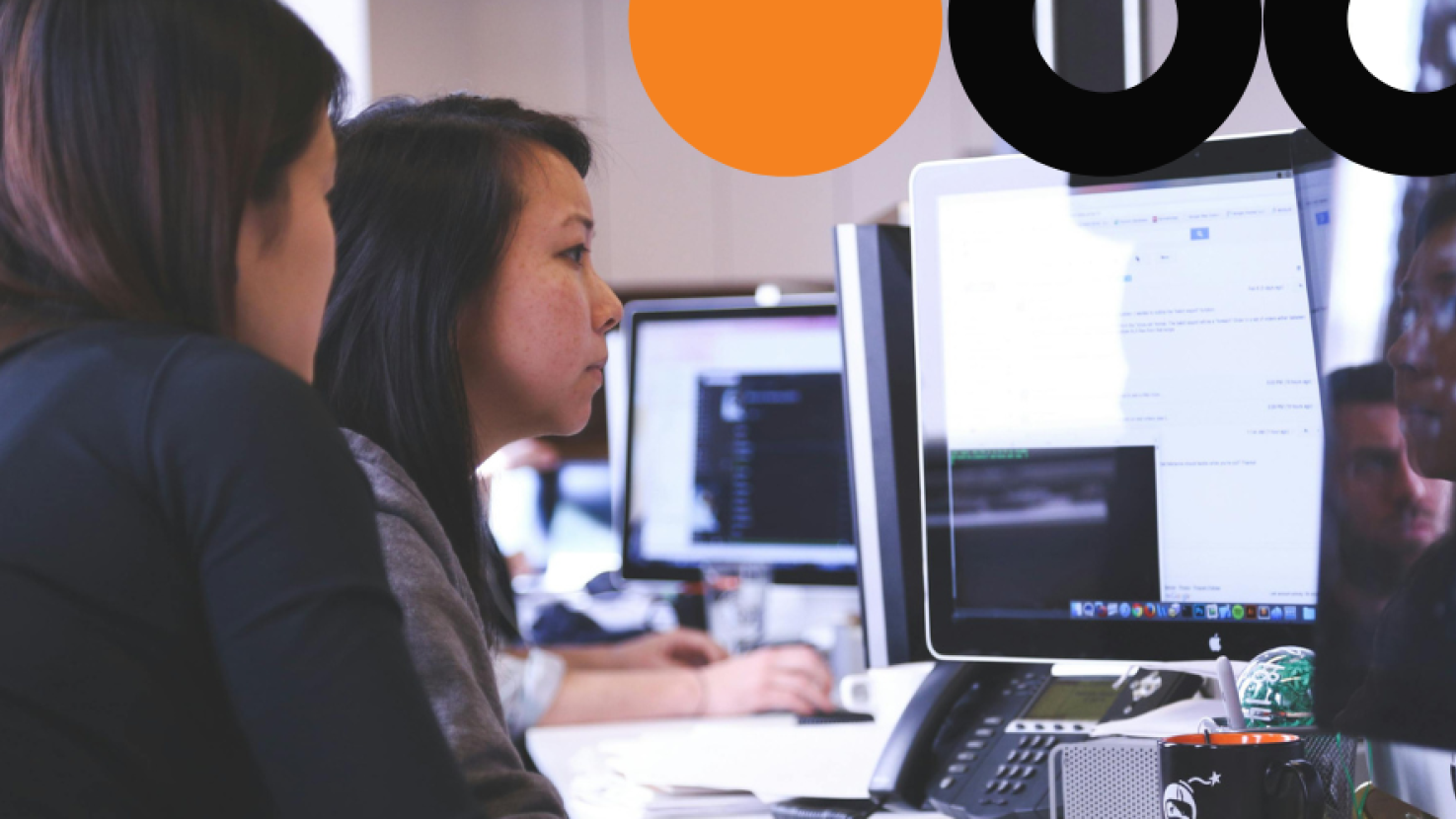
Ten years ago you often heard people say that we were in the subscription economy. Today you’re more likely to hear about the data economy, with data-driven products working away in every corner of our lives, helping us choose what we watch, the route we drive to get to work, whether we need to take more steps to reach our daily goal, or informing us that there may be a fraudulent charge on one of our financial accounts.
Although we have been building data products for at least 30 years, the pace has accelerated since ChatGPT brought Generative AI into the hands of everyday users. You can say without exaggeration that every business today is to some degree a data business. People are collecting data, have access to more data, and are storing and analyzing it more cheaply and efficiently, giving rise to the generative AI boom today.
Thinking holistically about the data you’ve got and who else may pay to use it in a product can yield great results. For example:
- Reddit content has been licensed for large language models
- By organizing and continuously updating all the recipes they’ve published, The New York Times has created an app that millions are happy to pay $5 a month to use
- Qualtrix, a customer experience platform focused on the Net Promoter Score, sells benchmarking services based on their status as the leading platform for customers who collect this information
Whether it’s traditional, structured data or unstructured content like research and research reports, companies are evolving from serving data to their own customers to licensing those data feeds into other platforms and datasets to reach broader audiences.
To give you a sense of the scale of today’s data ecosystem, consider that the VC company FirstMark’s latest MAD market landscape – MAD standing for Machine Learning, AI, and Data – has exploded from 1,416 companies in 2023 to more than 2,000 in 2024.
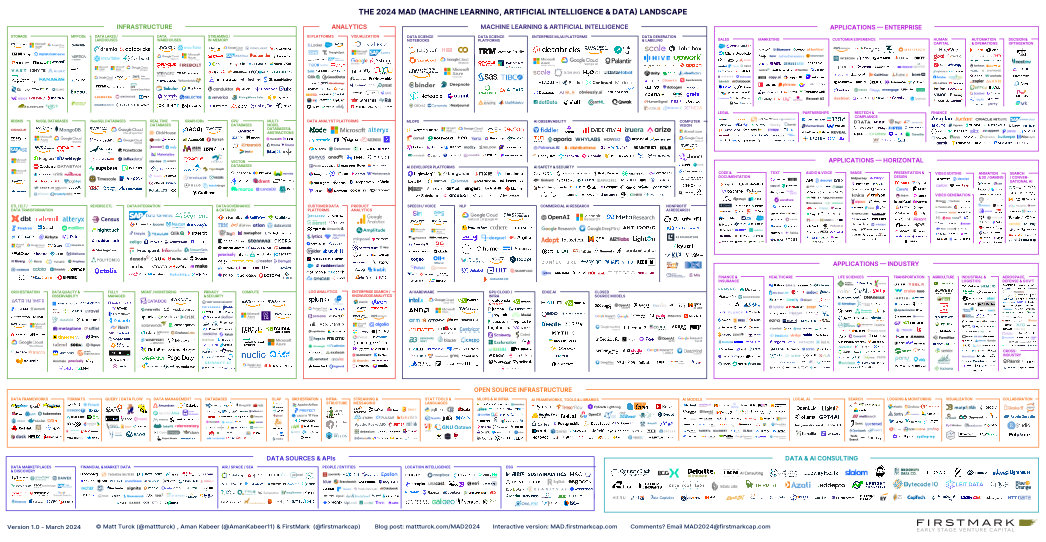
In 2015, most MAD companies fell under the category of infrastructure, analytics and applications. Now big data has enabled ML and AI, both of which have been around in some more rudimentary form for decades.
Statista estimates the global data analytics market will grow from $240B in 2021 to over $650B in 2029 creating opportunities, especially for nontraditional data providers.
Building a better data product
Data leaders are rightly trying to get the most value from their data by productizing it, but they can hit a number of barriers or challenges, which are often linked to the size of the datasets under their control. Based on my professional experience developing data products, here a few best practices to consider as you set out to build data products for your organization:
- Listen to your customers to identify new uses for your data. Listening to your customers to see how your data is being used is another way to identify new value propositions to sell your data. As an example, I recently talked to a software company that helps CIOs manage their IT spend, collecting data about how enterprises are spending on IT. In talking to their CIO customers to understand how the expense data gets used within their company, leaders in the software company discovered that there also is a ready market of Chief Financial Officers or Procurement leads who would value benchmarking data about how other companies of similar sizes are spending on IT.
- Determine what data you have. Data often lives in silos across businesses, especially acquisitive businesses, limiting the ability to identify and use complementary data. A good first step is just understanding what data sources are available to help identify which may create value if integrated.
- Clean and normalize your data. This is often the biggest challenge to getting data to market, because it takes time to map large datasets and make the information consistent across them. (e.g., reconciling different street addresses: 1000 Fifth Avenue or 1000 5th Ave, or company names: “IBM” or “International Business Machines”).
- Know what you can do with your data. Even if you have a license to use another data provider for internal purposes, you may not have licenses to use some of the datasets you acquired for external purposes. With data you can use, you must follow all the regulations governing its use. Whether it’s personally identifiable information, MNPI, or health records, there are many restrictions around what can be made accessible and for what purpose.
- Make your data easy to use. Your data must be well structured, of course, but you also need to think about how to make it easy to access and share. This generally includes delivering the data via tools that enable customers to visualize the data as well as tools that allow customers to access the underlying dataset to combine it with their own data, through APIs, or data marketplaces. Using common identifiers such as CUSIP numbers, DUNS numbers, etc. whenever possible will also make your data more accessible for customers.
Divesting to invest
Learning how to build data products that last requires striking a balance among a few different dynamics. One is customization. If you’re small or just starting out, you’re trying to confirm product-market fit by selling to whoever will buy, and you’ll do customization in order to get to those customers. As you expand and identify high-potential areas of future growth, there comes a point where you have to reassess the capabilities within your product. You can’t carry them all forward with you as a business. You have to prune your product offering in order to compete effectively and grow. Basically, you have to divest to invest. You may lose one or two customers along the way, but if done thoughtfully, you will gain much more by freeing resources that can focus on higher value datasets.
Determining what to build in your data product also requires a customer-centric ear. Listen to what customers say about your product but also watch how they use it and try to understand the ecosystem within which they are working.
For example, Google Maps started with simple navigation. By understanding that one pain point of travelers is finding local food, gas, shopping along their route, they were able to integrate this data into the user experience, driving value and saving time for millions of users.
Customers may not be explicitly asking for these things but if you’re a good listener you can surface their concerns and act on what really will drive value – whether they define value as time savings, increased revenue, reduced expenses, or decreased risk.
Your running data product punch list
This (by no means exhaustive) list touches on the kinds of questions you should be asking as a data leader when it comes to the products you develop and enhance:
- What’s the trade-off between the highest-value, lowest-effort thing you could do with your data product, and how could this drive your prioritization?
- Have you reaffirmed your data product priorities in the past quarter? When will you reassess this during the next quarter?
- Have you excluded any constituents in your company (Sales, Marketing, Legal?) who should have a say in what goes into the content or management of your data product?
- Have you built a culture of trust and humility that empowers your team to be candid about what’s working and what’s not working – to change your product to better align with the customers you serve?
- Do you see new opportunities to pull together products you’ve been selling individually in a way that can amplify the impact for customers?
- Are you communicating product changes among data, product, technology, and sales to ensure they are well communicated to customers and support customer retention?
Finally, remember that everything is relative with a data product. More content is not always better if you have to decrease coverage. Timely is not always better if you have to reduce quality. Playing and winning in the data product economy requires a level of vigilance and strategy that is matched to managing the complexity of what can feel like a whole new world.