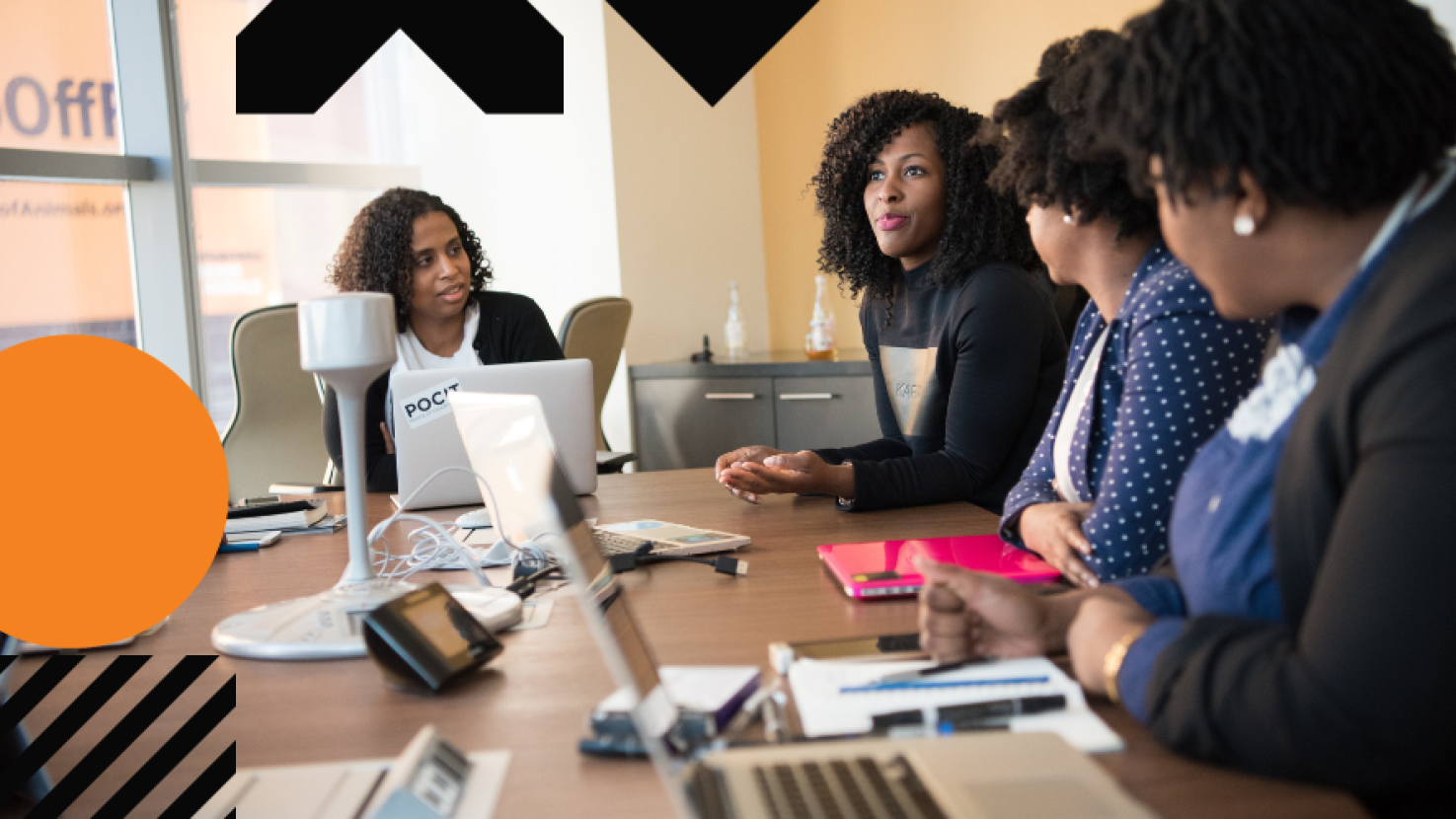

Driving Toward a Higher State of Data Analytics
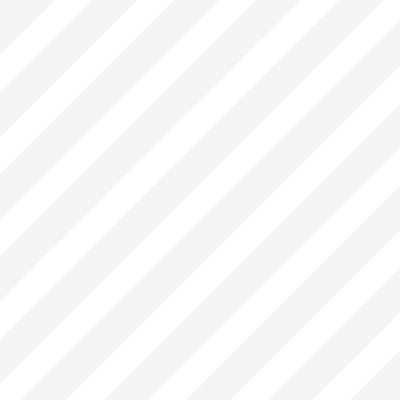
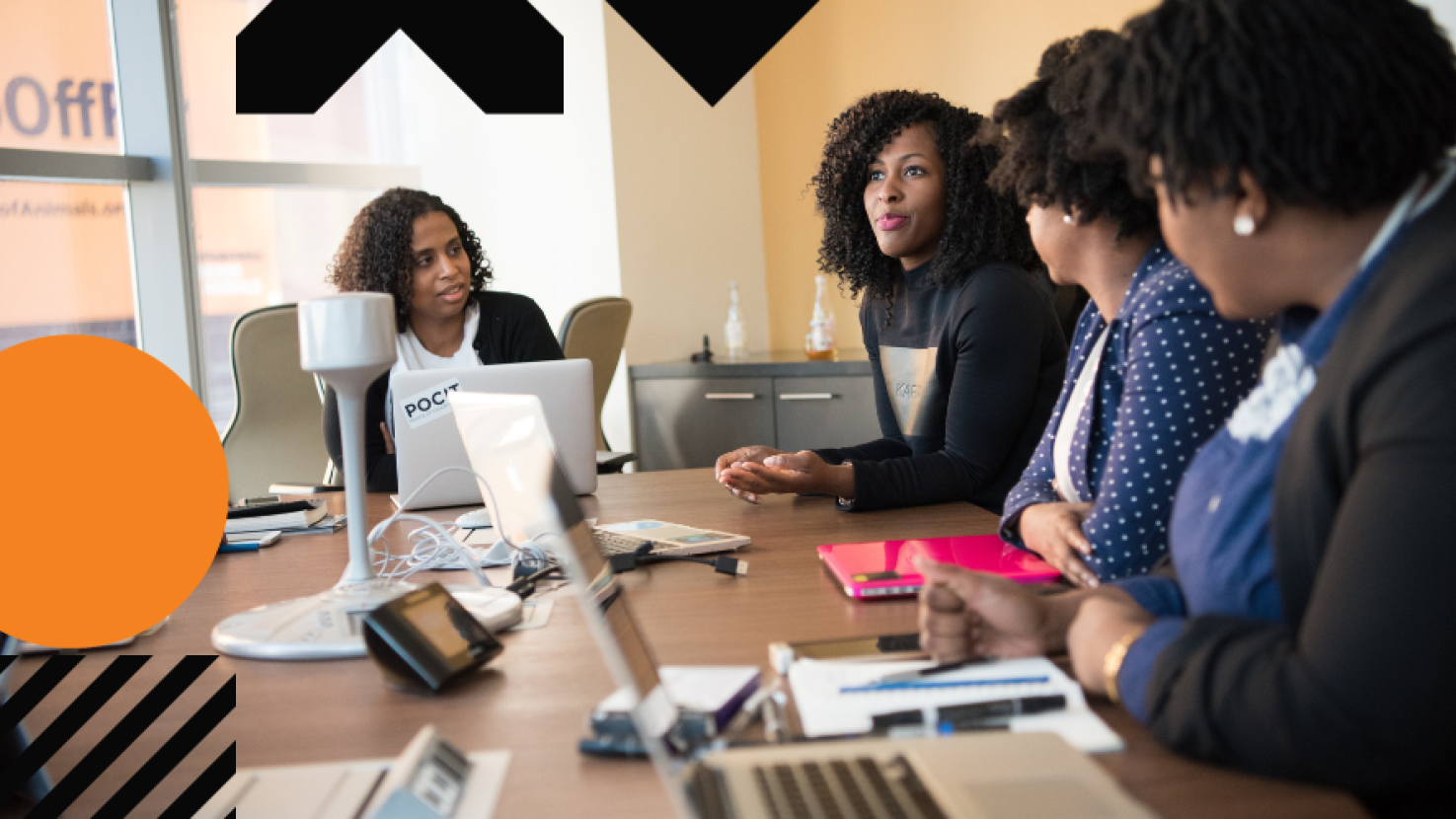
I hear a great deal of talk about GenAI among my network of data leaders. That’s to be expected. There’s a lot of hype, and getting natural language responses with ChatGPT is certainly impressive the first time you use it. For all the talk, though, only a small percentage of data leaders I know are all in on AI or have defined a clear path to realizing business value from it.
I see their caution as wise. Before anyone makes a move toward embedding AI in their business, it’s critical to have the right data strategy embedded first. A lot of organizations need to take Step One and Two up the data maturation curve before suddenly jumping to Steps Five and Six and wondering why AI isn’t doing anything for them.
As a Chief Data Officer, my impulse is always to go to the data strategy and determine where the state of data analytics is in a business unit or organization. Until the basic building blocks are in place, it’s impossible to get to topics like trust and business value.
Data strategy imperatives
If I had to choose three areas where data strategy must be strong for analytics to succeed, I’d suggest the following:
- Get your data together. I think it’s safe to say that most organizations don’t have data in a single repository. But to advance as a data organization, you need access to a single source of truth that is available to everyone that needs it. That’s a challenge, since some of those data sources may be on prem, in the cloud, or distributed across different locations. Data may sit with third parties based on engaging at a time when no one blinked an eye at sharing data. Or there may not be a protocol to gather data other than manual extract and upload.
An interface layer becomes critical here, as it creates an accurate, authoritative, and single source of truth in your data. That’s the first imperative in any data strategy, to create that quality foundation. After that, using tools like AI, machine learning, or data mining can be built on top.
- Focus on data quality. Low data quality is a non-starter for data analytics. If you’re fishing around in poor-quality data and can’t scale across different data sources, your analytics will be equally flawed.
Low quality could mean:- missing data
- poor discipline in collecting data
- limited understanding of how data is stored, which creates access challenges
- flawed data dictionaries, which means a KPI as simple as ROI can become a cause for extended debate
- Work from the business case. One practical reason to get data centralized and sorted is that you’re in much better standing when a business user requests access to data based on their belief that they’ll work out what to do with it as soon as they get their hands on it. In truth, if you don’t know the value you’re trying to get from data, you’re going to spend a lot of time just fishing around and hoping. So always insist on defining a business case to solve, whether it’s to sell more, save money internally, or improve a specific framework. That’s the proper order of things, starting with the end in mind.
The CDO as strategic intermediary
The role of a CDO as a leadership position is to both guide your team forward to talk to the business and guide the business forward to talk to the data team. Goal: realize the best value. If a misalignment exists between your data strategy and the business goals, you should start with the business goal. What is the business trying to achieve? Is it trying to achieve minimizing costs? Is it in a growth phase? What’s important to the business? For its part, the business should have identified its priorities.
The data strategy should then underpin these goals, be an enabler for them, and be written with them explicitly in mind. And the data leader should be laser-focused on aligning the strategies.
This strategic alignment should become clear as you scale analytics across the company. The four pillars of our data strategy were organized around the business goal of our Beyond100 strategy towards electrification and carbon neutrality by 2030. They are:
- Governance, which is primarily about improving data quality
- Data Cloud, which focuses on our data stack and how it ingests, transforms, and provides data to users
- Enablement, which means working with each area of the business to enable them to get more value out of dat
- Data Dojo, which is our bespoke data training program for staff at any level. It begins with Data 101 (White Belt), which not only allays data fear and teaches a common language, but uncovers use cases for data we may not have considered. It then allows staff to proceed up through six more “belts” to Data Professional, who are distributed throughout the business
Putting GenAI to Work
This leads me back to my opening point about GenAI and data strategy. As analytics and AI converge, the quality, governance, completeness, and access to data rise straight to the top of the agenda. How prepared is your organization in these areas?
Despite the growing awareness of its energy consumption and cost, GenAI is clearly the next evolution in computing. In its emerging co-piloting applications, it will help nearly all of us become better at nearly everything, in our personal or work lives. Like wireless technology, it will become more widespread and more embedded, often in ways that we may not even sense right away.
As data leaders, the onus is on us to make sure we have a strong business case for using AI, and that it’s not a solution looking for a problem (remember Blockchain?). As long as we can articulate what we’re trying to achieve, we can use AI in whatever form to get to desired outcomes quicker and more efficiently. But we still need human inspiration and creativity to get the most from it.
So don’t be afraid to test and learn to realize your business objectives. Just make sure you’ve got the right data foundation to build from first.
A note from the DLC: As companies rapidly embrace AI and realize its benefits, trust must be their top priority. And to instill trust in AI, they must first instill trust in the data that powers it. Check out the full Salesforce State of Data Analytics report here.