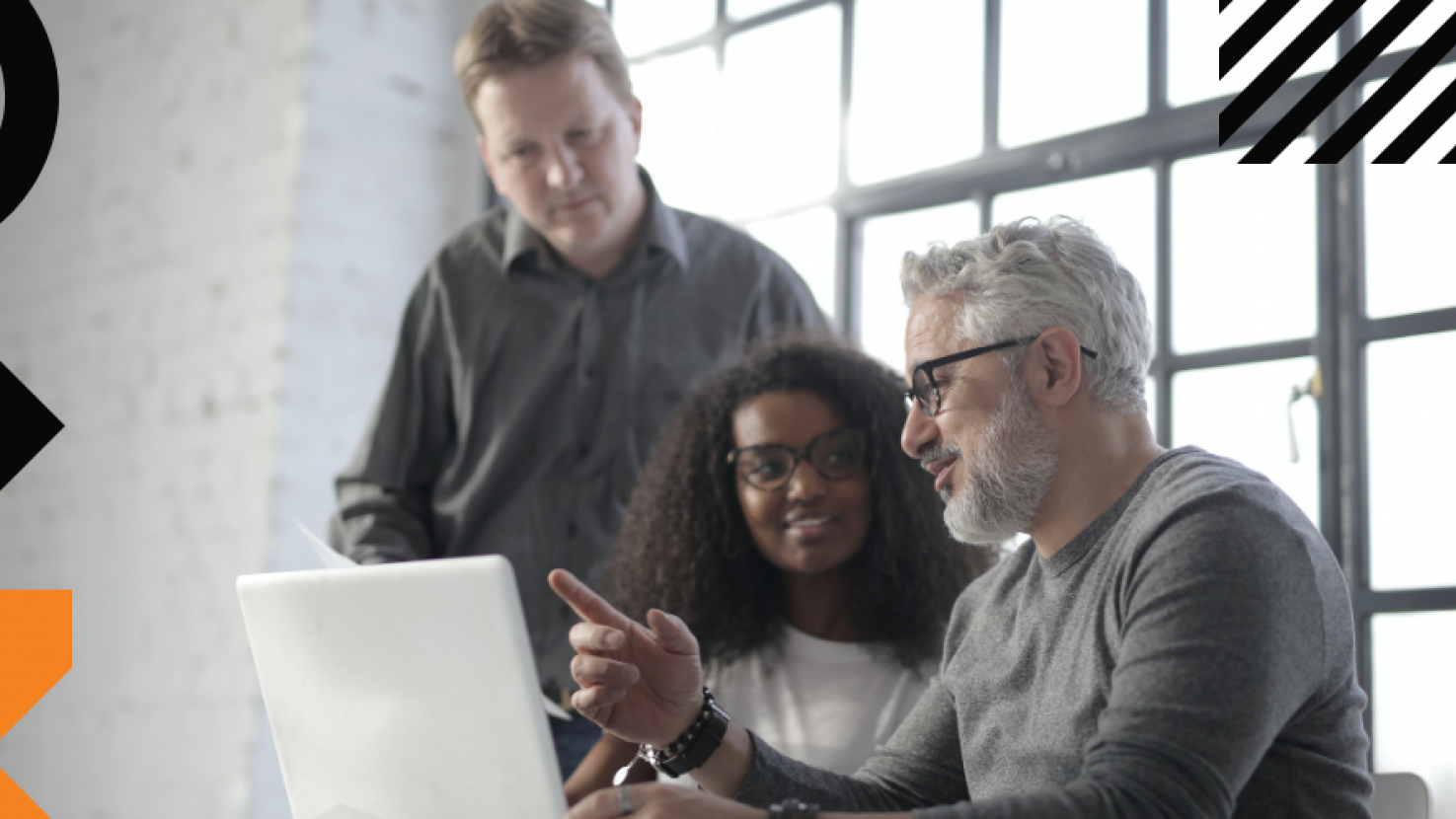

The Mid-Career Possibilities of a Data Career
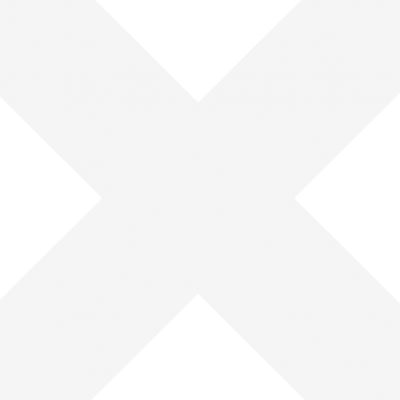
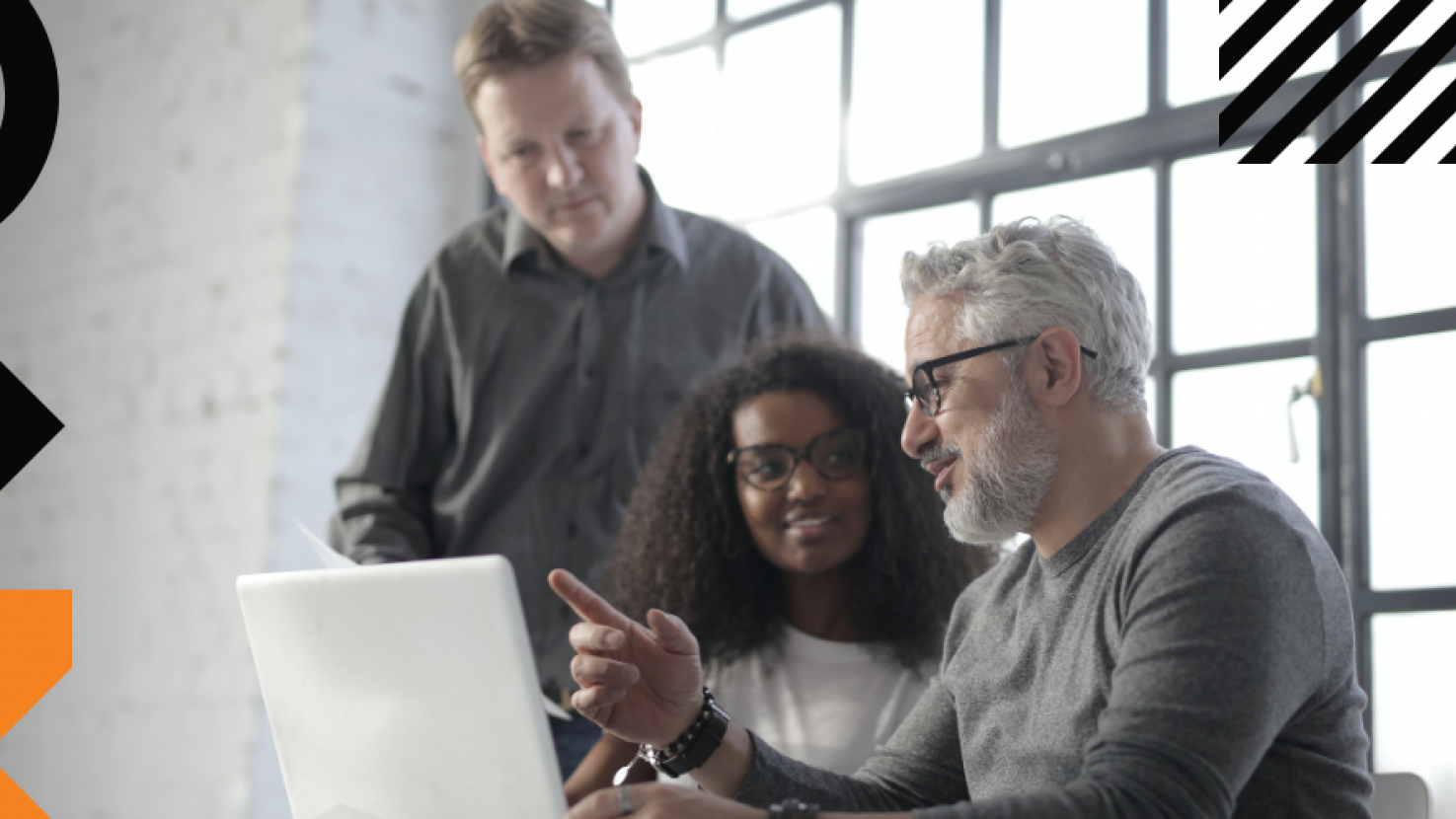
Until quite recently, declaring to an employer or career coach that you wanted to pursue a job in data would have returned some blank stares. The options for a formal career path in data only appeared in the past several years. We both have lived through this evolution, since one of us double majored in math and computer science, then did a PhD in applied math, while the other started with a PhD in Accounting and pursued quantitative research in the field.
Yet momentum has begun to coalesce around data science based on its promise to solve tough real-world strategic problems for organizations. Another helpful dynamic we’ve seen is the increasing number of mature students – working professionals who’ve wanted to pivot – joining the ranks of those pursuing careers as data scientists. That’s why we’re both passionate about ensuring clear alignment between what’s being taught in the academic space and what’s needed and expected from industry.
An ever-expanding menu
The spectacular rise in data since the 2010s, both in volume and type, has required new techniques to analyze it all at scale and as close to real time as possible. That’s why the prospects for anyone entering the data field today are both bright and exciting, whether your tastes and abilities lean toward natural language processing, graph analytics, computer vision, or business intelligence.
Barriers to entry have fallen as well. You no longer have to be a computer scientist and a hardcore coder to pursue a career in data. You no longer even need to enter a traditional course of training thanks to options like Coursera and Udemy. You only need a computer, an internet connection, the time, and the willingness to learn.
Entry points abound, too. A strong mid-career data scientist could emerge from:
- Accounting
- The armed services
- Econometrics
- Marketing
- The sciences: biology, math, physics, chemistry, or social science
- Statistics
Finally, your career path can flex depending on how left-brained you’d prefer your day-to-day job to be. For example:
- If you become a traditional data scientist, you need a strong understanding of the fundamentals of the math and statistics that underpin it
- If you’re becoming a data engineer, you need to understand software engineering paradigms and have a strong coding focus
- If you’re keen on becoming a business intelligence or visualization expert, math and statistics aren’t as imperative as storytelling creativity and a strong understanding of psychology and how people think, both of which are essential
Change applies to the field – and to you
As much as the war for strong data talent continues, beginning a career in data science requires an appetite for change. As digitization and automation continue to sweep through industries, you will have to adapt your skills, reskill, and upskill to maintain strong and enjoyable employment. That’s simply a reality in our AI and ChatGPT world.
In addition to the right technical skills, here are five skills you will need to call on as you start down the path in data. Incidentally, it never hurts to call out these skills on your CV as well:
- A love of solving problems. This includes framing, analyzing, and reporting out on solutions
- Curiosity and a results focus. In other words, your interests should extend beyond just theory and research
- Flexibility and agility. As conditions shift, since they inevitably do, rigidity in views or approaches tends to backfire in data science
- Teamwork and collaboration. Both are absolutely vital. This includes the ability to work well and efficiently in teams
- An openness to continual learning. This means you’re never satisfied with domain knowledge only as it exists today
Speaking of openness, don’t overlook older, established companies in industries such as healthcare or packaged goods in favor of the latest tech startup as you launch your data career. The government is another area rich in opportunity for data science professionals. Both may surprise you with the sophistication of their systems and the wealth of data they’ve collected, often for many years. And your learning curve may not be as sharp or steep as it’s likely to be in a tightly resourced startup.
Ultimately, success in a data career is a function of the ability to navigate with and adapt to a culture, whether that’s a corporate culture, a data culture, or both. And whether you come at it head-on, as you can do today, or via a slightly more wayward path, as both of us have done, it’s an endlessly rewarding pursuit whether you’re considering a mid-career switch or looking at the final act of a long career.