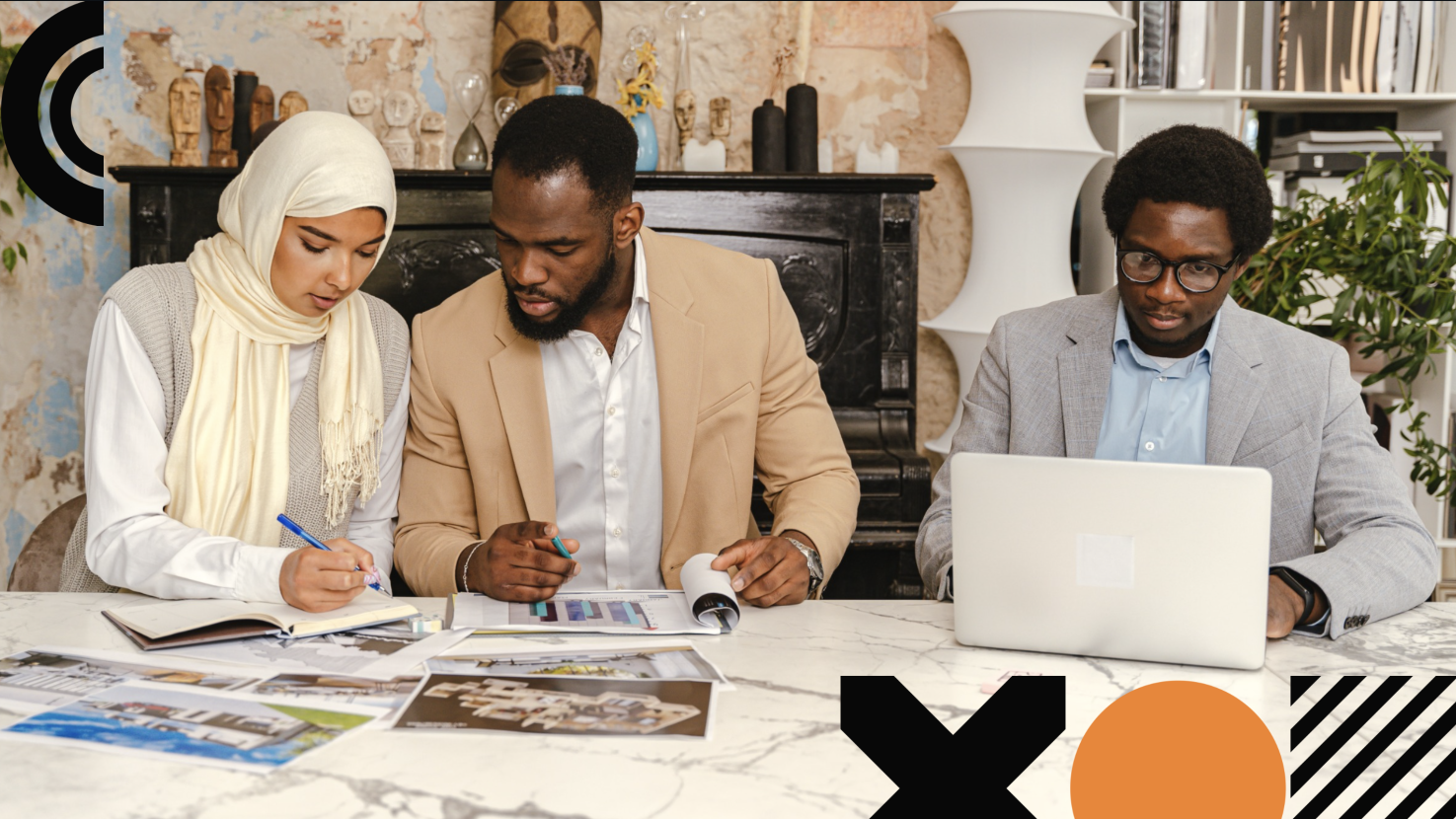

The Three Key Data Literacy Personas
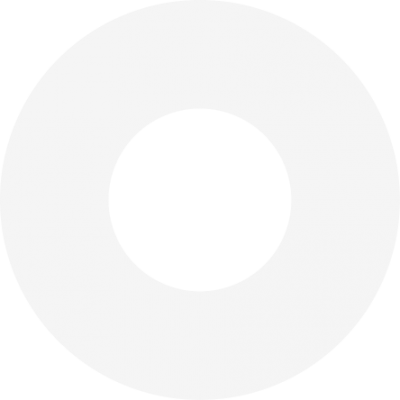
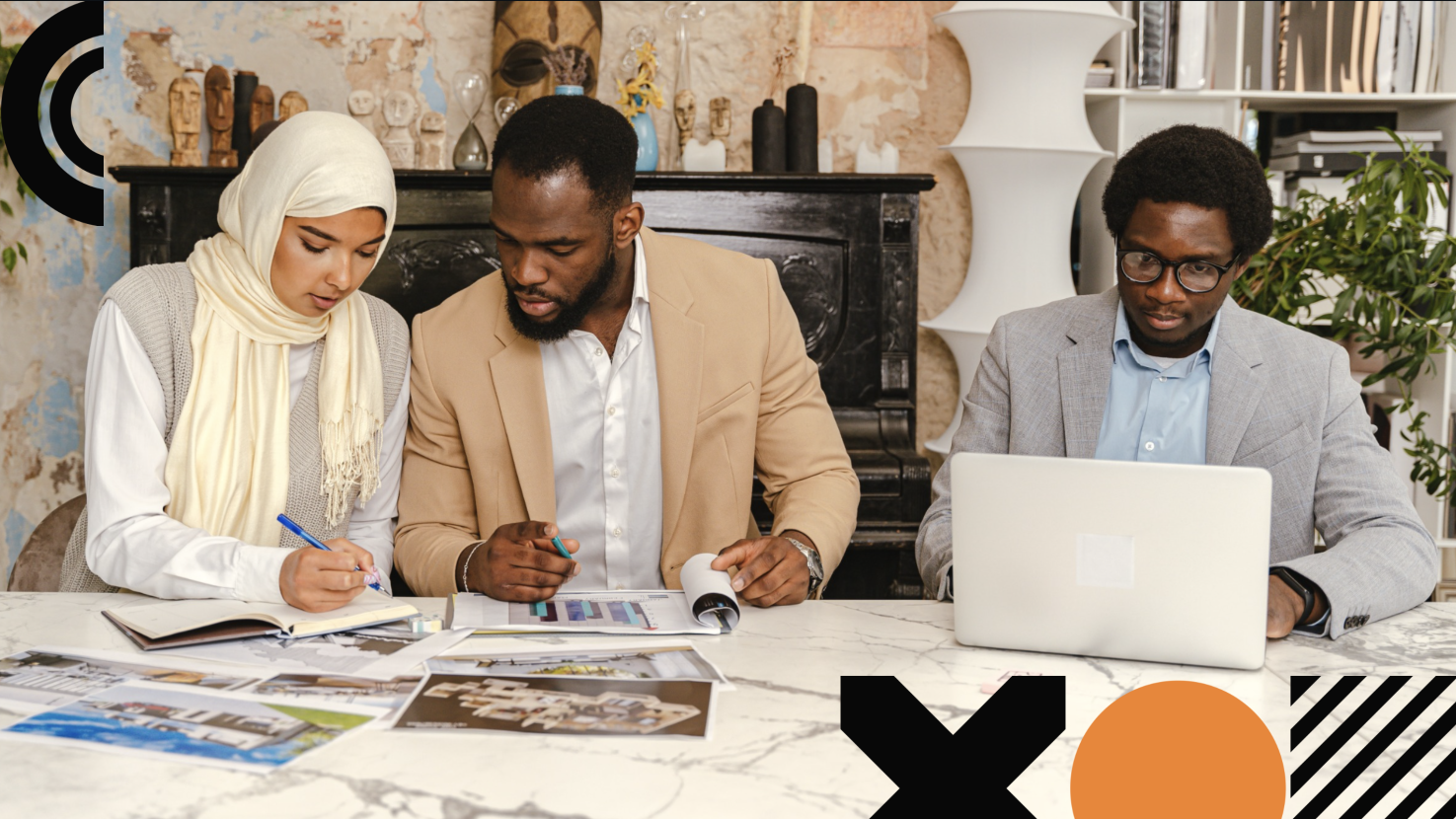
In the first article of this series, I addressed how data literacy programs help to upskill the workforce as part of broader digital change. I also touched on how it unlocks collaboration across different teams, and ideally leads to self-service, which frees up bandwidth for data scientists to focus on tough problems.
All this begs the question, exactly who are we solving for with these programs?
One of my Data Lodge clients came up with a great way to talk about the data literacy personas we should keep in mind:
- People who use data in their day-to-day role
- People for whom data is part of their role (their “minor”)
- People for whom data is their role (their “major”)
As data leaders, we need to build data literacy as a shared foundation across these personas. As I think about it:
Literacy means a shared way to read, write and communicate with data in context. When you have data literacy, you feel less fear or vulnerability around data. It is both a foundational work skill, and a life skill, that you are aware of, and comfortable with.
Fluency is the next layer on top of literacy, which can be thought of as creating with data and developing different dialects of the language. With fluency comes confidence, whether you’re a citizen data scientist or a business analyst.
Mastery sits above fluency, and involves taking literacy to the level of coaching and helping others. Data mastery leads to conviction in knowing that you can teach data literacy as part of your role, whether you’re a data engineer, AI modeler, or Chief Data Officer.
Fostering shared language, intentionally
Thinking about literacy led me to develop the ISL model, which stands for Information as a Second Language®. This is an obvious riff on the English as a Second Language (ESL) concept. I like to use this model because it makes the idea of language explicit. When people can approach data as a language they learn, in the same way an English speaker learns German or Spanish, it brings down the fear and potential vulnerability. Unless you live in Germany, no one criticizes you if you don’t speak German. In fact, if you are learning the language of data, it’s something to be celebrated. Think of it as something you’d put on your LinkedIn profile.
Just as a language has different dialects of its vocabulary, the same is true with data. If you're in Finance, HR or supply chain, or you’re in a certain division or business unit, you specialize in a different dialect of data. While data can feel complicated sometimes, segmenting it into vocabulary, dialects, and skills can help to simplify that complexity.
Beyond myths and misconceptions
In my work coaching and advising data leaders, six data literacy misconceptions come up frequently. To close out the second article in this series, here are things to be on the lookout for as you engage with different audiences:
- Data literacy is just about training. Clearly, it’s about continuous learning and long-term practice.
- Data literacy only touches on internal data and statistics. In reality, it’s about the diversity of data and methods.
- Data literacy is just about visualization and storytelling. That is a part, certainly, but far from the whole.
- Data literacy is about making everyone a junior data scientist. That might be nice, but people have other jobs to do in organizations.
- Data literacy is not just a work thing. In fact, data literacy is a life skill and a work skill.
- Data literacy isn't relevant to experts. Actually, by scoping data literacy to include business acumen, data acumen and analytical acumen, there is always something to learn. Even the most advanced data scientist isn't an expert in all areas of business acumen.
Regardless of which term you choose to describe your program – data literacy or fluency or acumen, make sure you’re ready to dismiss these myths and misconceptions. Dispelling them is critical because if you don’t, they will interfere with building engagement as your team moves toward their goals of data literacy, data fluency, and data mastery.
So how do you bridge the gap between theory and practice in a data literacy program and serve all three personas equally well? That’s the topic of the final piece in this series.